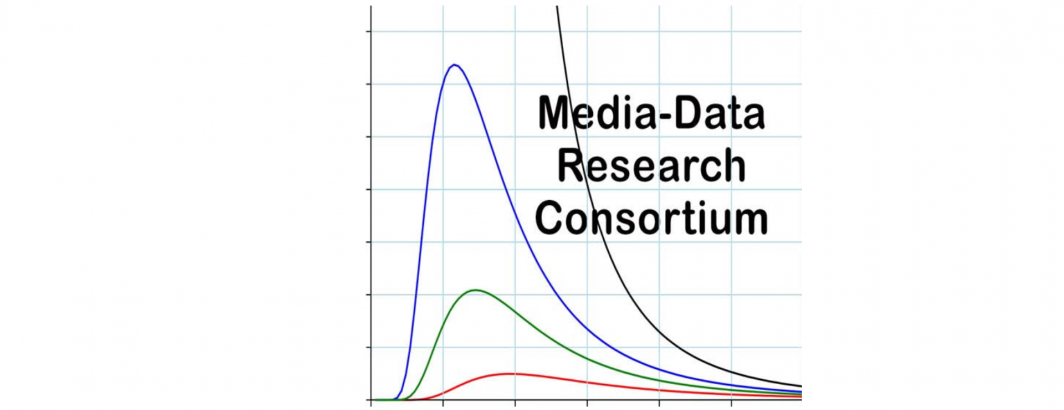
We are tremendously excited to announce that the Media-Data Research Consortium (M-DRC), whom GDELT has been working closely with to analyze television news, was awarded a grant of $1,560,000 worth of Google Cloud Platform credits under a Google Cloud COVID-19 Research Grant to support “Quantifying the COVID-19 Public Health Media Narrative Through TV & Radio News Analysis.”
How does the COVID-19 narrative differ across television, radio and online news and across outlets? Is COVID-19 being covered differently than past disease outbreaks like Ebola or Zika and what can we learn from those communication efforts that could help inform public health communication about the current pandemic?
To help answer these critical questions, the M-DRC is working with GDELT to use Google’s Cloud Video and Cloud Speech To Text APIs to non-consumptively analyze selections of the Internet Archive’s Television News Archive and Radio News Archive in a secure research environment, analyzing in total more than 4.9 million minutes of television news across 1,113 days 2009-present and 2.5 million minutes of radio since the start of this year to create an open set of non-consumptive annotations to enable public health communication research on how the COVID-19 pandemic has been communicated to the public and how those communicative efforts compare with the pandemics of the past decade, including Cholera, Ebola, E. coli, Measles, MERS, Salmonella and Zika and a portion of the opioid epidemic.
In terms of television stations, the totality of BBC News London, CNN, MSNBC and Fox News are being processed from Jan. 1, 2020 through present, while a selection of days from CNN, MSNBC and Fox News surrounding past disease outbreaks that received substantial attention on those stations since the start of the Television News Archive are being processed (dates in parentheses are the general boundaries of substantial television news coverage of the given outbreak on the three stations, not the dates of the outbreak itself):
- H1N1 (July 2009 – May 2010) (July 2009 is the start of the Television News Archive)
- Salmonella (August 2010)
- Cholera (October 2010 – January 2011)
- Ecoli (May 2011 – June 2011)
- MERS (April 2014 – May 2014)
- Ebola (July 2014 – January 2015)
- Measles (January 2015 – February 2015)
- MERS (June 2015)
- Zika (January 2016 – October 2016)
- Opioid Epidemic (March 2017 – April 2017)
- Measles (March 2019 – May 2019)
- COVID-19 (January 2020 – present)
As part of earlier pilot work we’ve already processed the broadcast evening news programs of ABC, CBS and NBC from July 2009 through present.
Each broadcast is analyzed through the Cloud Video API with Label (identifying the objects and activities depicted), OCR (onscreen text), shot/scene detection (camera changes) and ASR (speech recognition) features enabled. All of the results can be interactively searched and visualized using the AI Television Explorer. The visual annotations for each broadcast are also being made available for download as JSON files and through BigQuery as part of the Visual Global Entity Graph (see its description for the specific fields it makes available) while the ASR transcripts are used to generate subsecond captioning alignments for the AI TV Explorer interface.
What are the kinds of research questions this new data will make possible? Here are just a few examples you can try right now (date ranges vary based on what we’ve processed to date):
- CNN’s use of its COVID-19 Dashboard which has appeared up to 9 hours a day since March 20. [View Live Graph]
- Onscreen textual mentions of deaths, showing CNN mentions it far more than its peers, while MSNBC and BBC have largely dropped their mentions since the George Floyd protests, despite BBC being a UK outlet. [View Live Graph].
- Depictions of “personal protective equipment” (from facial coverings to sports helmets to eye protection) since Apr. 3, with BBC and MSNBC depicting them the most. [View Live Graph]
- How crowds disappeared from the news in mid-March, reappeared with the George Floyd protests and remain slightly elevated. [View Live Graph]
- Onscreen textual appearances of Donald Trump’s social media handle “realDonaldTrump” (such as displaying one of his tweets on air) shows Fox News relies more heavily on presidential communications to drive its coverage. [View Live Graph]
- Tracing on-air appearances of specific tweets, such as this August 3rd Trump tweet about school reopening, showing it was even aired on BBC and that Fox News covered it the least. [View Live Graph]
- Using BigQuery to compile a chronology of the doctor interviewees telling the COVID-19 story. [View SQL Queries & Spreadsheet]
- How bookcases first began to appear on CNN regularly in mid-March. [View Live Graph]
- Appearances of bookcases in the background of BBC, CNN, MSNBC and Fox News coverage since Apr. 3 as they became a theme of COVID-19 coverage, showing BBC and MSNBC favor them the most. [View Live Graph]
- How CNN began relying heavily on Cisco’s WebEx for its remote interviews since March 9. [View Live Graph]
Radio news is another major form of information in the United States that has remained absent from most analyses of pandemic news coverage. Given radio’s heavy presence of personality-driven programming, we are especially interested in whether radio news systematically differs from television coverage, as well as how it varies geographically across the United States.
We will be analyzing the following ten radio stations from Jan. 1, 2020 through present through the Cloud Speech-to-Text API and creating ngram word frequency histograms for them, making them available for search in a modified version of the TV Explorer and are exploring creating entity recognition datasets for them as well:
- BBC Radio 4 FM
- BBC World Service
- KFNX 1100 AM (Phoenix, Arizona)
- KGO 810 AM (San Francisco, California)
- KKNT 960 AM (Phoenix, Arizona)
- WBAI 99.5 FM (New York City)
- WDBO 96.5 FM (Orlando, Florida)
- WGBH 89.7 FM (Boston, Massachusetts)
- WAPI 1070 AM (Birmingham, Alabama)
- WFLF 94.5 FM (Panama City, Florida)
In addition to these new datasets created by the Media-Data Research Consortium’s Google Cloud COVID-19 Research Grant, GDELT itself also has numerous web-derived datasets that are highly relevant for studying the pandemic, spanning more than 3 trillion datapoints over 152 languages.
The Global Knowledge Graph offers a realtime metadata index of worldwide textual online news coverage in 65 languages spanning more than a billion articles and was used to send one of the earliest external alerts of the pandemic on December 31st of last year. The Global Geographic Graph geocodes location mentions in global news, allowing instant mapping using tools like Carto’s BigQuery connector. The Global Entity Graph has analyzed more than 103 million of these articles in 11 languages through the Cloud Natural Language API, constructing a dataset of more than 11.3 billion entities ready for trend analysis. The Global Quotation Graph tracks quoted statements in 152 languages, making it possible to track the statements of elected officials, health officers and others over time to understand shifting narratives on everything from vaccine concerns to misinformation. The Global Relationship Graph that can be used to track worldwide English-language media statements regarding everything from reaction to mask mandates to vaccination concerns. The Global Frontpage Graph tracks the homepage links of more than 50,000 news websites every hour, tracking the stories editors around the world are prioritizing. Turning to the visual world, the Visual Global Knowledge Graph has analyzed more than half a billion news images stretching back half a decade through the Cloud Vision API, analyzing the pandemic’s visual portrayal.
As officials progress from encouraging lockdowns to complex layered reopenings to therapeutics to potential vaccines, they must convince an increasingly divided and exhausted public to embrace science-based adaptations to their individual behaviors. Resistance to such public health guidance is an inevitable response to a public witnessing science playing out in realtime, with ever-changing guidelines and assurances against a backdrop of myriad voices issuing contradictory recommendations. As the pandemic progresses from medicine to messaging, health officials need to understand the competing public narratives into which they will be communicating.
Infrastructure projects learned long ago that communicating complex science to a skeptical public through statistics is rarely successful. Instead, gaining public acceptance requires listening to local concerns, understanding the public narrative and information environment and crafting tailored messaging for each constituency. Opponents of a wind farm might be concerned about bird migration, noise, EMF, etc. Successfully easing these concerns requires understanding these public narratives, which in turn requires the ability to look across the multimodal news spectrum from online to television to radio.
Over the coming weeks you will begin to see these new television and radio datasets begin rolling out, with weekly releases as processing completes, so keep an eye on the blog for each latest release!
All of the data being produced is open and available either for download or through Google's BigQuery platform. If you have any questions about the data or additional datapoints and analyses you think would be useful to your work, we'd love to hear from you! Email us at Roger Macdonald and Kalev Leetaru.
The Media-Data Research Consortium is a fiscally sponsored project of the 501(c)(3) Community Iniatives. It is dedicated to creating collaborative opportunities for scholars, archivists, journalists, data scientists and other researchers to conduct noncommercial public interest research on diverse media to enhance information integrity and address the scourge of disinformation. Of particular interest, in this time of global pandemic, is facilitating insights into the propagation of health and related social/behavioral messaging in media to inform critical citizen decision making.
The Media-Data Research Consortium is also collaborating with another outgrowth of the TV News Archive, Bad Idea Factory’s TV Kitchen, funded by the Knight Foundation. The new TV Kitchen is an open source tool to derive data from local TV streams–starting with captions, and in the future political ads, chyrons, talking points, and more. TV Kitchen tools, in conjunction with Consortium resources, will provide opportunities both to collect and analyze local TV programming.
The Media-Data Research Consortium is committed to being rigorously mindful of ethical considerations in the application of computational analysis to television, radio and other digital news archives. It is paramount that, as we serve the public good, we are careful to protect civil liberties. We believe that underlying all our efforts we must welcome considerations of unintended consequence. Consortium-related research will attempt to foster working models of how machine intelligence can serve critical public interests while being guided by the evolving work of a number of efforts, like the Asilomar AI Principles, Google’s AI Principles, the Stanford Institute for Human-Centered Artificial Intelligence and the Ethics and Governance of AI Initiative at the Berkman-Klein Center and the MIT Media Lab.