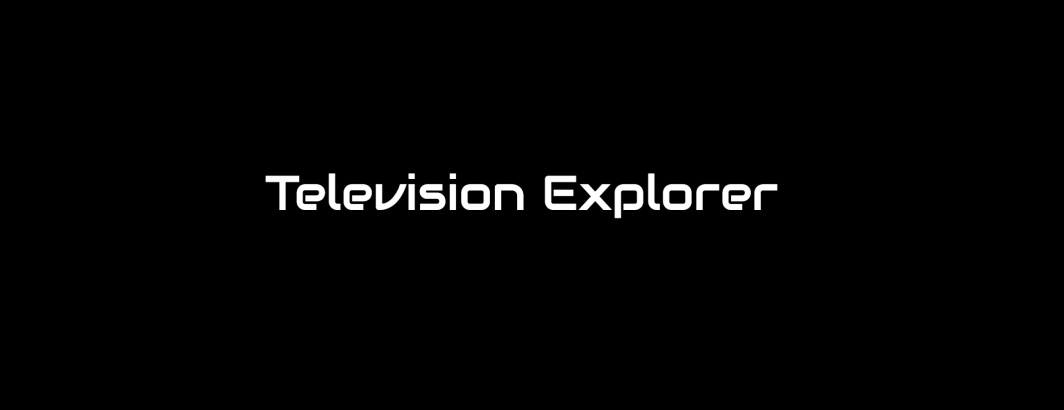
Most analyses of television news coverage explore differences in media attention across topics, over time, between networks or all of the above. How much attention is Donald Trump receiving compared with Alexandria Ocasio-Cortez? Is Trump getting less attention this month compared with a year ago? Is MSNBC paying more attention to the Mueller story than CNN?
All of these questions can be answered through GDELT’s Television Explorer interface to the Internet Archive’s Television News Archive.
Yet, sometimes the most powerful answers can come not from the attention paid to issues, but from how those issues are framed, whether the way they are expressed overall, over time or across networks. Issue framing can be explored by using the Top Clips list in the Television Explorer and processing into a word histogram, known as an ngram set, with unigrams (single word histograms) and bigrams (two-word phrase histograms) typically offering the most interesting results. For example, FiveThirtyEight took this approach in comparing the differing language used by CNN, MSNBC and Fox News to describe the Cohen hearings.
By breaking the 24-hour television news cycle into a sequence of discrete 15 second windows, we are able to understand the media cycle’s attention in a uniform and directly comparable way. Taking the set of 15 second clips that mention a given keyword and turning them into word and phrase histograms of those words/phrases that appear in immediate context with a keyword allows us to do for media what the famous Google Books ngrams did for books: allow rich exploration of linguistic trends in a non-consumptive fashion that enables powerful analysis without granting access to the underlying fulltext.
Using these word histograms, researchers can run topical, sentiment and other forms of linguistic analysis across the immediate context of every mention of Trump and Mueller over time, allowing detailed analysis of how the narrative surrounding them evolved, while being fully respectful of the underlying content by limiting access to non-consumptive means that do not grant access to any meaningful portion of the original captioning.
In short, by running a keyword search and compiling a list of all of the 15 second clips that mentioned the keyword, then turning those clips into a daily histogram of words and two-word phrases, we can enable rich linguistic analysis of the narrative surrounding the keyword without making available the underlying fulltext.
Thus, we are excited today to announce two new non-consumptive ngram datasets compiled from the Television Explorer that allow you to explore how CNN, MSNBC and Fox News have covered the candidacy and presidency of Donald Trump and how they have covered the Mueller investigation.
The Television Explorer was used to generate a daily list of all matching 15 second clips mentioning “Trump” since June 15, 2015 (the day before his announcement of his candidacy for president) through March 24, 2019 for CNN, MSNBC and Fox News and the same for “Mueller” since May 17, 2017 (the day he was appointed as special counsel) through March 24, 2019.
Under the hood, the Television Explorer is actually a wrapper around the GDELT TV API 2.0. To generate these two mentions archives, the Television Explorer was used to craft the two respective queries and confirm that they yielded less than 3,000 results per day (the limit of the TV API) which was then used to compile the list of mentions for each day. These mentions were then converted to unigram and bigram datasets by day/station, generating a histogram of all of the words and 2-word phrases appearing on each station on that given day across all of the 15 second clips that mentioned the keyword “Trump” or “Mueller.”
In all, 2,226,028 total 15 second clips were found across the three stations that mentioned Trump, along with 218,135 clips mentioning Mueller, which were converted to the ngram datasets.
To create the ngrams, each clip was lowercased and split on spaces. Punctuation occurring at the end of a word was removed except in cases of punctuated acronyms (any word matching the regular expression “[a-z]\.[a-z]\.$”), in which case the period was preserved, to allow for words like “u.s.” for example. Bigrams were computed by running a 2-word rolling window across the clip, resetting at the presence of punctuation other than a punctuated acronym. Thus, a string like “trump said that. yes. he did say that, I’m sure.” would be converted to “trump said” and “said that” and “he did” and “did say” and “say that” and “I’m sure” as the full set of bigrams. No normalization or stemming is performed, so “mueller” and “mueller’s” are counted as two separate words.
Both the 1gram and 2gram files are tab delimited, one word/phrase per row with the following format:
- Day. Records the day the ngram was computed from, at day resolution.
- Word. The unigram or bigram.
- Total Mentions. The total number of times the word was mentioned that day across all of the matching clips. If a word appears multiple times in a clip it is counted multiple times here.
- Total Clips. The total number of distinct clips containing the word one or more times. If a word appears multiple times in a clip, it is counted only once for this field.
To assist with normalization and filtering low-volume days, a “totals” file is also available for each station that is tab delimited and has the following format:
- Day. The date in day resolution.
- Total Words. The total number of unigrams/bigrams found that day.
- Total Clips. The total number of distinct clips found that day.
A small number of shows were accidentally excluded from the Archive’s original index and thus are missing here while they are being processed for inclusion, but this dataset includes all of the shows currently indexed by the Television News Archive and thus the Television Explorer.
Think of this new dataset as a template of what can be done with the Television Explorer to move beyond simple attentional analysis towards rich narrative assessment, from topical and sentiment analysis to linguistic and framing trends. While the current dataset combines all shows on a given station together into a single daily ngram, imagine using the same approach to filter to specific shows, comparing coverage of a topic across a station’s key media personalities or comparing opinion versus news coverage.
In 2014 we released our first whole-of-television look at the emotions of television news, following it in 2016 with an even larger and richer emotions dataset, demonstrating the power of looking at television at scale. Stay tuned as next month we will be releasing a 10-year non-consumptive ngram dataset that will make it possible to explore the broad contours of the topical and emotional landscape of television news over the past decade using your own ngram analyses!
We’re incredibly excited to see what you are able to do with these powerful new ngram datasets and hope they will inspire you to think of new kinds of rich new non-consumptive linguistic analyses of the news!
TRUMP
- CNN: 1-Grams
- Fox News: 1-Grams
- MSNBC: 1-Grams
- CNN: 1-Gram Totals
- Fox News: 1-Gram Totals
- MSNBC: 1-Grams
- CNN: 2-Grams
- Fox News: 2-Grams
- MSNBC: 2-Grams
- CNN: 2-Gram Totals
- Fox News: 2-Gram Totals
- MSNBC: 2-Grams
MUELLER
- CNN: 1-Grams
- Fox News: 1-Grams
- MSNBC: 1-Grams
- CNN: 1-Gram Totals
- Fox News: 1-Gram Totals
- MSNBC: 1-Grams
- CNN: 2-Grams
- Fox News: 2-Grams
- MSNBC: 2-Grams
- CNN: 2-Gram Totals
- Fox News: 2-Gram Totals
- MSNBC: 2-Grams