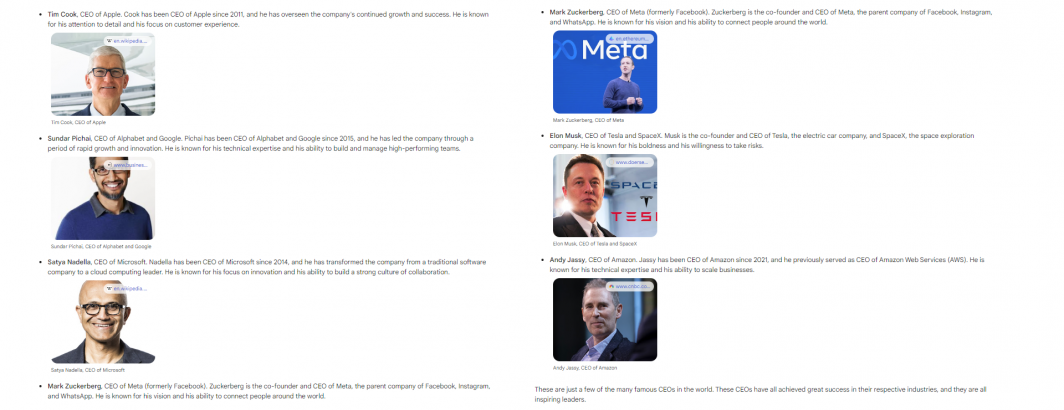
Last week we explored just how devastatingly innate gender and racial bias is in both LLM generative models and LLM-based embedding models. Most shockingly, when asked to rank a set of LLM-generated hypothetical biographies about a collection of CEOs by relevance to the search "ceo", the LLM embedding model ranked the white CEOs as the most relevant, followed by African American male CEOs, followed by white and African American female CEOs intermixed at the bottom. In other words, in a real-world semantic search application (nearly all of which today are based on embedding models) or a real-world "generative search" application (which typically rely on embedding models for their external knowledge stores), the embedding model will surface information about white male CEOs first, prioritizing those results above those of women.
Yet, recall that a closer look at the underlying fictional biographies generated by ChatGPT for each gender/race combination exhibited marked differences in how they described the fictional CEOs. White male CEOs were described in terms of their professional track records running successful large companies. White women "broke the glass ceiling." African American men and women "overcame adversity" and were "inspirations" to other underrepresented groups, though men were more likely to be described in terms of professional accomplishments than women. In other words, in the eyes of ChatGPT, male CEO biographies focused on their work accomplishments, female CEO biographies (and African American male CEO biographies) focused more on how they got there and their personalities.
That raises an existential question: just what is a CEO?
Is a CEO defined only by what they do at work once they receive the title? Or on their entire lives up to that point? This has long been a point of contention in the pre-generative world of executive biographies, where those written of men tend to focus on their accomplishments, while those written of women more often emphasize details like their fashion choices and family lives. In other words, when an embedding model is asked to find articles most relevant to the concept of a "CEO" what should it emphasize? Articles about CEOs' professional accomplishments as CEO or those about how they became a CEO or some mixture of both?
The real underlying question is whether embedding models have truly learned innate gender and racial biases from their training data or whether the differing focuses of the biographies generated by ChatGPT are the underlying issue. Perhaps embedding models are gender and race-neutral and the reason we saw such stark gender/race sorting is that the embedding model picked up on the male stories emphasizing leadership at work, while the female stories emphasized their path to the CEOship and the models associate CEOs with leadership at work, not the path to the corner office. This would in turn suggest that even if further work is done to debias models, they will still yield biased results simply because of the way executive biographies are often written. In turn, this suggests that one avenue for debiasing would be to step back and consider just how precisely we define a "CEO".
The reality is that embedding models encode starkly clear gender biases, with the mere appearance of a female pronoun typically resulting a significantly different association for stereotypical professionals like doctors vs nurses, etc.
Another key challenge is that models rely on digital knowledge: the internet. What happens if we ask Bing Chat for "who are some famous CEOs?" Just one solitary woman appears (Indra Nooyi).
Bard offers two versions: one has no women:
And one has a solitary woman (Meg Whitman):
What if we don't care about famous CEO? Let's just ask "Who are some CEOs?" in a brand new session (to avoid cross-contamination with our request for famous CEOs). The results aren't much better:
Nor for Bard:
For both Bing and Bard, we can see that the sources they are relying upon tend to emphasize male CEOs. Given that we cannot look inside their internal ranking systems, we can't answer the most important question of all: while it is likely that many of the lists of CEOs on the internet are male-centric, to what degree are Bing and Bard's ranking systems elevating those lists above ones that are more gender-neutral and to what degree are their models' encapsulations of what a "CEO" is driving these rankings?
In the end, embedding models encode very clear biases, but moving beyond those biases is not as straightforward as it might seem, forcing a reckoning with myriad deeper non-technical questions like just what a "CEO" is: someone who holds the title or someone whose entire life story lead them to that title?