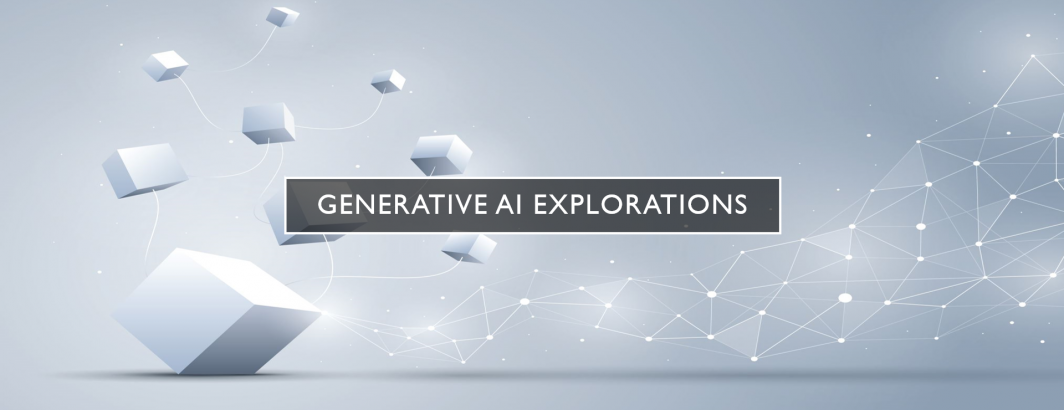
Current embedding models used in Retrieval Augmented Generation (RAG) architectures, from the most simplistic DAN-based to the most advanced multimodal LLM-based, suffer from a wide range of challenges including existential bias, NMT poisoning, the limits of multilingualism, knowledge cutoffs, oversensitivity and lack of steerability, failure of coherence and comprehension and myriad other limitations. Over the coming weeks, one of our newest series will explore how to improve the current state of embedding-based LLM external knowledge store integration approaches such as RAG, examining both embedding-specific approaches and evolving approaches such as cascading and interwoven systems (embedding + LLM intermixed at multiple stages), boosted and hybrid systems (LLM + embedding + classical neural and statistical models), the use of graph models (especially multigraphs) versus embedding retrieval, and some extremely exciting forthcoming architectures that replace the current LLM-centric model with a far more advanced generative pipeline.
Stay tuned for the first of this series in the next few weeks!