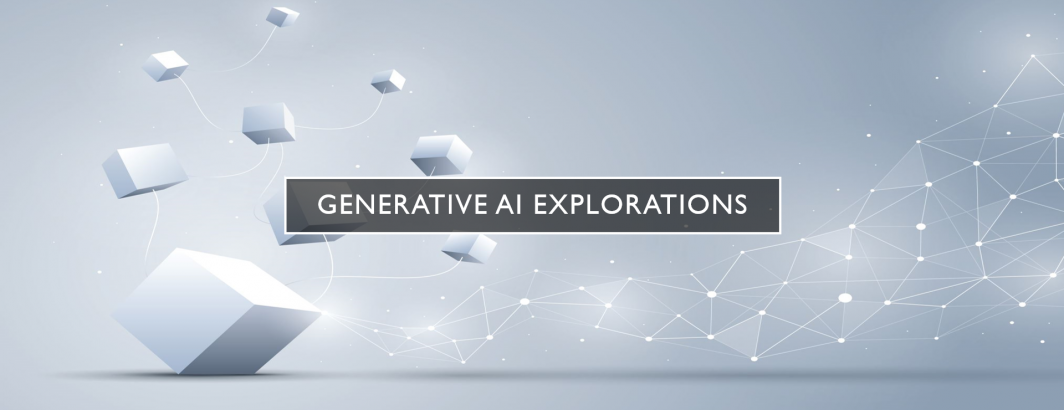
One of the strangest aspects of the generative AI revolution is the vast disconnect between the hype expressed in public and the growing concern recognized in private about the limitations of genai systems. In public, nearly every company in the world and a growing number of governments are touting only the hype and hyperbole of generative AI, putting it forth as the solution to every societal ill. Yet, behind closed doors, those same companies and governments acknowledge its massive limitations, from bias and brittleness to hallucination and false confidence.
Generative models are infusing the digital world with a new era of racial, gender, linguistic, cultural and other societal biases that are now being established as the "rules of the road" for the modern world. Men are strong leaders pioneering the future, women are followers whose role is to sit quietly in the background and encourage their husbands, men are intelligent and women beautiful, LGBTQ relationships don't exist, minorities are not successful, non-European religions are evil, certain nationalities are terrorists, biometrics work best on certain skin tones and so on. While in the past there was a brief moment of reflection and recognition in the AI community of the vast harm these biases cause, the generative AI rush has thrown all of this work away, placing these biases now at the apex of the rules that guide our modern generative world. As our own work has demonstrated again and again, these biases are getting steadily worse and there seems little interest from the global AI community to address them as evidenced by the failure of red teaming efforts to flag even the most egregious of these issues and the lack of interest or corrective action in our own engagements across the field.
From a technical standpoint, current generative AI systems are plagued by an extensive array of existential limitations like hallucination. As awareness of hallucination has grown, the industry and the surrounding army of AI-centric consultants has reacted not with acknowledgement and mitigation efforts, but rather with false promises and wrongful hype claiming magical solutions. Influencers tout "one weird trick" solutions to their followers, major consulting firms openly tout "magical fixes" to governments for astronomical sums and companies brush aside concerns in the interest of reducing labor costs with AI worker replacements. Yet, behind closed doors, those same sources acknowledge the existential limitations that hallucination places on the real-world application of generative AI. The same companies that tout instant fixes to governments and push them to deploy to their most critical tasks are the first to generate internal rules to limit genai use to non-essential applications. In fact, many of the most vocal advocates of generative AI that publicly tout its deployment across their entire organization are in fact actually engaging merely in "AI washing" in which they integrate a minor capability somewhere out of their critical workflows or utilize a simplistic tool like an LLM-powered embedding so that they can claim to be an "AI first" company. Intriguingly, a growing number of companies are deploying generative AI in the same way they deployed large data analytics: *after* the decision making process. An executive makes a decision and a generative AI system is used to justify that decision by cloaking it in a veil of superhuman AI decision making.
Rather than brush aside these issues, companies need to more directly acknowledge and address the limitations of current generative architectures.