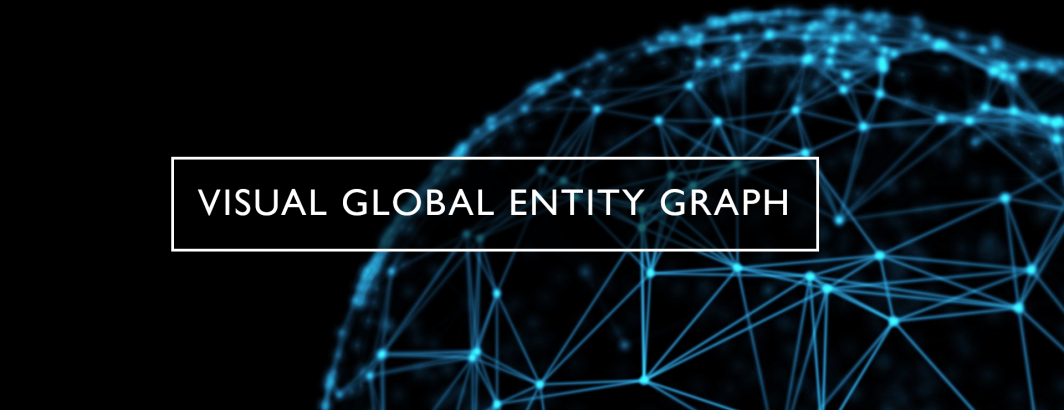
Through the Media-Data Research Consortium (M-DRC)'s Google Cloud COVID-19 Research Grant “Quantifying the COVID-19 Public Health Media Narrative Through TV & Radio News Analysis" a rich array of open datasets have been created that catalog the global narrative around Covid-19 across online, television and radio news covering text, still imagery, spoken word and motion video formats. Using these massive datasets, how might creative visualizations help public health officials, policymakers, journalists and scholars understand the narrative space around the pandemic in ways that could help advance public health messaging?
The global Covid-19 pandemic has yielded a chaotic information environment filled with outdated statements and advisories, conflicting recommendations, legitimate scientific disagreement and willful and inadvertent falsehoods. The result is a complex chaotic contested cacophony of information confronting the public in which accurate, inaccurate and deliberately false information all swirl together. From masking and social distancing compliance to vaccine hesitancy, the public health implications of this “infodemic” are severe. Similar information environments can be seen around myriad other contested topics from climate change to voting, showing the importance of being able to look across a topic at scale.
What might it look like to visualize the rapidly evolving narratives surrounding a topic like Covid-19? Imagine the public health opportunities opened by being able to automatically extract from news coverage a list of common vaccine hesitancies (from side effects to concerns about fertility to outright falsehoods like microchips) and visualize those into a narrative map such as a graph structure that showed how those concerns were related in terms of cooccurrences in news coverage or semantic connections? Or perhaps mapping geographically or temporally? What about identifying and mapping conspiracy theories? Or automatically identifying “contested narratives” in which a visualization could help surface claims or topics that seem to be emerging in opposition to one another in clusters in the media (for example one set of media outlets arguing for masking while another cluster argues against)?
Particularly interesting are visualizations that can help journalists, policymakers and public health officials make sense of the media environment around Covid-19, especially actionable areas like vaccination, masking, social distancing, etc. The goal of such visualizations would be to help sift out the high-level patterns in media coverage, for example identifying common topics or claims found in vaccine hesitancy coverage and visually summarizing it. In areas where scientific knowledge is still evolving, such as vaccine efficacy against virus variants/mutations, understanding the media environment around the topic and what is being said in the news about this can help guide public health messaging by managing expectations and better communicating uncertainty and what is known at the moment.
Other interesting visualizations could be mapping public statements about a topic such as masking. Using the Global Quotation Graph, one can easily track statements that contain the keywords “mask/masks/facial coverings/n95/kn95/etc” and visualize in some kind of clustered timeline how the discussion around masking evolved in a given country/language/government/geography to help better understand public messaging around a topic more holistically and the information environment into which health officials must message.
Essentially pretend you are a public health official or policymaker or journalist confronted with an endless firehose of news coverage flooding the public with chaotic contradictory information and you’re trying to A) understand what the public is seeing so you can amplify or counter-message it and B) learn from it such as novel vaccine hesitancy concerns. What might such visualizations look like?
How can visualization help address these needs?
Through the Media-Data Research Consortium (M-DRC)'s Google Cloud COVID-19 Research Grant and GDELT's online news monitoring, we have datasets covering text, imagery, radio and television news. User-friendly interfaces that don't require analyzing large datasets include the TV Explorer for closed captioning search, the TV AI Explorer to search a subset of AI-analyzed television news 2020-present and Web News search across machine translations of global coverage in 65 languages. Given the size of these datasets, it is often best to start with small subsets of the data. The two TV APIs are a particularly good place to start, since they can be used to understand trends, narrow a particular query, etc. Several small snippet datasets are also available.
Public health researchers looking for narrative datasets, students in information visualization courses, open data scholars looking for an interesting project and anyone else are encouraged to explore these datasets for ideas on how to visualize the Covid-19 media ecosystem. If you come up with particularly compelling examples using these datasets, we'd love to hear from you and feature your visualizations on our blog!