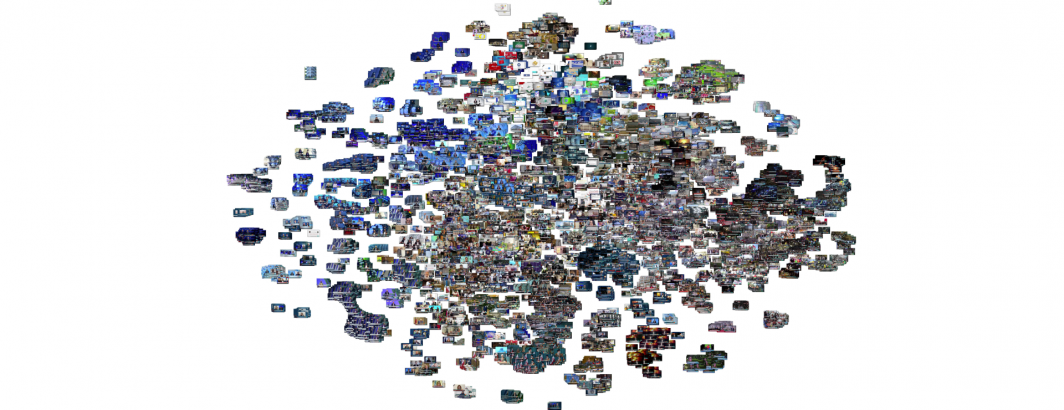
In a global world beset by an growing number of crises and conflicts, it is more important than ever for journalists and scholars to be able to look across the global narrative landscape to understand the public information environment being presented to the world's citizenry. Within the nations of the world, how are domestic television news media presenting global developments and what kinds of framings, contexts and visual storytelling metaphors and devices are they using? To explore this question in more depth, yesterday we examined how multimodal image embeddings could be used to understand the visual narrative landscape of an entire day of one channel of Russian television news. Today we repeat that analysis by visualizing an entire day of a single channel of Persian-language Iranian television news: IRINN.
Using the exact same code and workflow as yesterday, we use GCP's Vertex AI Multimodal Embedding model to compute embedding representations of one frame every four seconds from the complete 24 hours of coverage October 17, 2023 (in UTC timezone). The embeddings were then clustered and visualized using PCA (which attempts to preserve macro-level trends) and t-SNE (which attempts to cluster images more tightly to yield more strongly-defined micro-level patterns and clusters). You can see the final images below.
Whereas Russian television as captured by Russia 1 on the same day yielded a fairly stratified collection of tightly-defined clusters, IRINN coverage on the same day is far more diffuse, with much heavier overlapping of clusters.
PCA
t-SNE