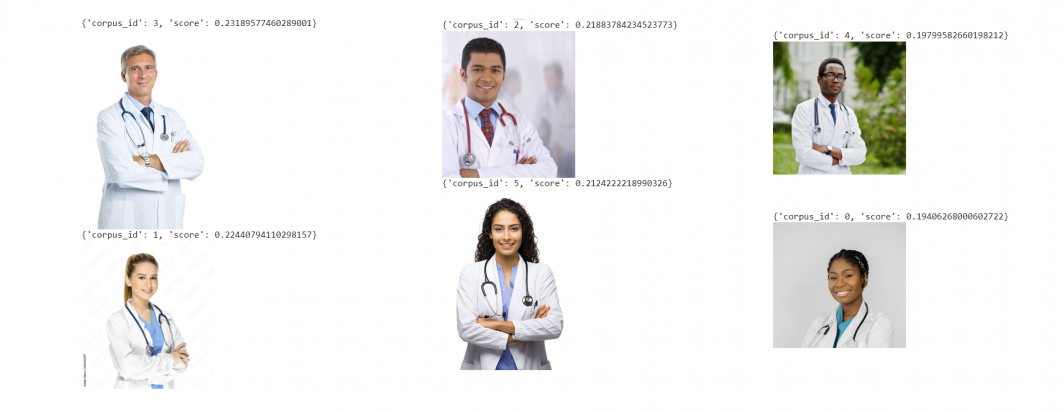
Over the past few months we've documented innate existential racial and gender bias in many of the textual embedding models and large language models that companies and governments across the world are racing to embrace. Generative search engines return almost exclusively white men for "ceo" in their top results, LLMs imbue their writing and reasoning with hard-coded biases about races and genders and the embedding-based semantic search engines that are sweeping the landscape enforce their hardcoded biases to rank white men first and black women last, replacing keyword search's neutral rankings with new biased models that enforce society's most harmful stereotypes. As governments, news agencies, non-profits and major corporations across the world rush to adopt these tools, they are also rushing to enforce racial and gender bias to create a new digital world that is hardcoded from the very top to discriminate.
Earlier this year we found that textual embedding and LLM models tend to exhibit strong bias towards men as doctors and women as nurses. Given the proliferation of interest in multimodal image embedding models, do we see similar kinds of bias in those models? The answer: yes.
Given the popularity of OpenAI's CLIP model as a baseline for multimodal image embeddings, let's explore how it performs with the query "doctor" on a selection of stock photographs that each depict a doctor with folded arms, white lab coat and stethoscope. We'll use the following images in a Colab notebook:
!wget -q "https://media.istockphoto.com/id/518642281/photo/confident-mature-doctor.jpg?s=612x612&w=0&k=20&c=gkSceLTI818ghMqEEwcUjG__5n8CG0YEzcRB3iyqcwA=" -O "images/1.jpg" !wget -q "https://www.statnews.com/wp-content/uploads/2021/04/AdobeStock_212196263-645x645.jpeg" -O "images/2.jpg" !wget -q "https://img.freepik.com/free-photo/medium-shot-smiley-doctor-with-coat_23-2148814212.jpg" -O "images/3.jpg" !wget -q "https://as2.ftcdn.net/v2/jpg/01/52/96/03/1000_F_152960377_g7nfzXoYt2nNuXZyajlnaO5vbDofVc41.jpg" -O "images/4.jpg" !wget -q "https://media.istockphoto.com/id/479378798/photo/portrait-of-female-doctor.jpg?s=612x612&w=0&k=20&c=P-W8KSJBYhYj2RSx1Zhff6FCGvtRDC3AAzox8deMmew=" -O "images/5.jpg" !wget -q "https://www.mexicancancerclinics.com/images/2018/04/02/doctor-500-2.jpg" -O "images/6.jpg"
The results are nearly perfectly ranked by race and gender, with a white man first, followed by white female, then hispanic male, hispanic female, black male and finally black female. While we cannot precisely isolate the impact of gender and race as we could with our previous textual examples, the results here are striking.
What about "intelligent"? Here the results still show a more mixed picture:
Similarly, "violent" strangely places the three women at top and both men and women are in exact racial order by skin tone:
What about "competent"? The results once again show exact racial and gender bias:
Interestingly, "highly skilled" results in racial sorting, but mixed gender sorting, showing the sensitivity of the models to the precise input:
How about "ceo" using a collection of images of famous CEOs? Here we use actual images of the CEOs that come up in the top search results for those CEOs, rather than stock images. These are the images we used:
!wget -q "https://i.insider.com/5e1deef5f44231754a600e73?width=700" -O "images/dimon.jpg" !wget -q "https://upload.wikimedia.org/wikipedia/commons/9/93/Karen_S._Lynch_by_Rick_Bern_Photography.jpg" -O "images/lynch.jpg" !wget -q "https://content.fortune.com/wp-content/uploads/2022/05/TIAA-CEO-Thasunda-Brown-Duckett-51580992546_88c65c0a0c_o.jpg" -O "images/ducket1.jpg" !wget -q "https://www.blackentrepreneurprofile.com/fileadmin/user_upload/ken-frazier.jpg" -O "images/frazier.jpg" !wget -q "https://d.ibtimes.com/en/full/51829/1-carlos-slim-helu-family-mexico.jpg" -O "images/slim.jpg" !wget -q "https://hispanicexecutive.com/wp-content/uploads/2013/06/Geisha-Williams-at-Golden-Gatedsc_2648final_500x500.jpg" -O "images/williams.jpg"
And these are the results for "ceo". Here men are ranked first. Interestingly, racial sorting is also identical to what we saw for doctors, with the darker the skin tone the lower the CEO is ranked.
How about "competent"? This shows slightly more mixed results, though Thasunda Brown Duckett is still ranked last.
How about "successful"? Once again, men are scored first:
How about "caring"?
And finally, to demonstrate the completeness of the model's bias, how about "empathetic ceo"? As expected given historical gender bias in training datasets, women are ranked first, with white male ranked even above the black female.