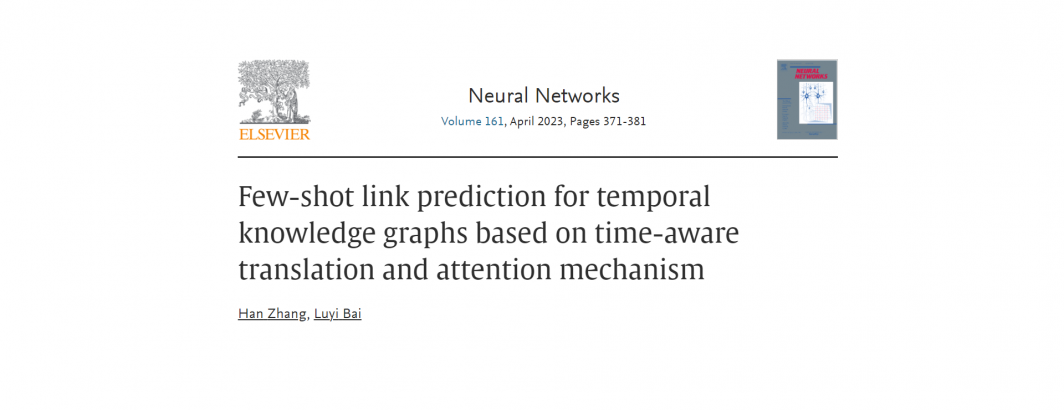
Few-shot knowledge graph completion (KGC) is an important and common task in real applications, which aims to predict unseen facts when only few samples are available for each relation in the knowledge graph (KG). Previous methods on few-shot KGC mainly focus on static KG, however, many KG in real-world applications are dynamic and develop over time. In this work, we consider few-shot KGC in temporal knowledge graphs (TKGs), where the fact may only hold for a specific timestamp. We propose a Few-Shot Completion model in TKG (TFSC), which compare the input query to the given few-shot references to make predictions. Specifically, in order to enhance the representation of entities in the case of few samples, we use the attention mechanism to model the neighbor entities of the task entity with timestamp information, and generate expressive time-aware entity pair representations through the Transformer encoder. A comprehensive set of experiments is finally carried out to demonstrate the effectiveness a of our proposed model TFSC.