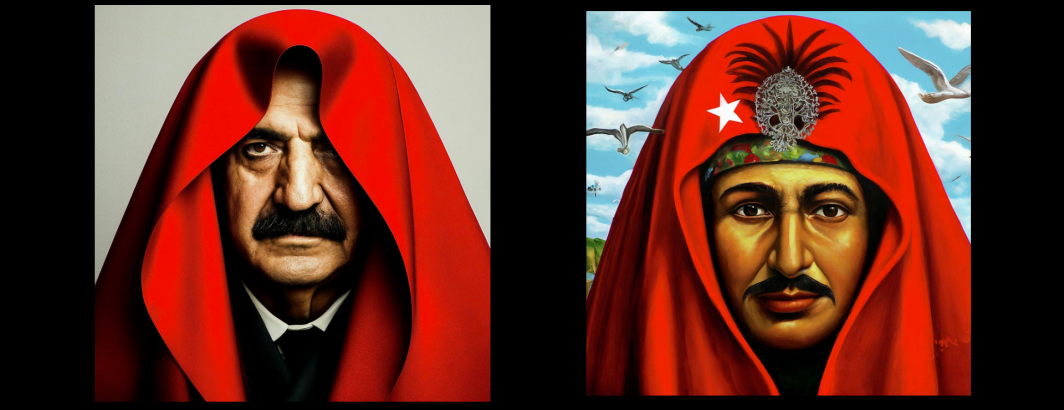
As we continue to explore how various generative AI offerings from different companies perform across global news coverage, one area of especial interest has been their systemic bias issues. Below is a summary of just a few of the existential bias themes we've observed across the various text and image generative models, embedding models and guardrails:
- Embeddings. When embeddings were first popularized several years ago, researchers quickly identified intrinsic gender, racial and cultural biases the models had learned from their training data. At the time there was a rush to attempt to mitigate these biases through a variety of methodologies. Unfortunately, that interest in correcting bias appears to have largely disappeared in the gold rush of generative AI and coincided with the existential return of all of those biases with a vengeance, with all of the major embedding models we have tested to date demonstrating significant latent biases along myriad dimensions: race, gender, religion, geography, language, dress, sexual orientation, presentation, national origin and myriad other dimensions of culture and society.
- Models. Textual models exhibit systemic bias throughout their performance. As multimodal models become the new GenAI frontier, they are exhibiting all of the same biases as textual models.
- Models hallucinate that Hispanic men must be tattooed and encode that dreadlocks are not acceptable business attire.
- Any video showing Joe Biden walking must depict him as stumbling around confused and asking for help.
- Any video of a woman in Africa with her hands held forward must depict her holding a child and food rather than merely gesturing to someone.
- Gender & Race In ChatGPT's CEO Stories & How Embedding Models Rank White Male CEOs First And Women Last.
- Geographic knowledge skews towards the West.
- Models have little contextual understanding of cultures outside historical Western European cultural practices.
- "Inspiring" images of Africa frequently depict National Geographic-style starving children, barren desserts define Saudi Arabia and a solitary tree represents Estonia. Models have immensely skewed visual representations outside of the US and Western Europe.
- Values, beliefs, lived experiences and cultural practices common to wealthy and largely white and male Silicon Valley communities define how the models "see" the world.
- How Zelenskyy and Ukraine are viewed as weak failures and Putin a strong successful leader due to the inability to prioritize recency in training data.
- Frozen-in-time context & inability to update their knowledge stores.
- Western bias in knowledge of public figures.
- Guardrails. GenAI guardrails have largely been developed by Western corporations and developers that are immersed in a fairly uniform values monoculture. This infuses itself into the guardrails that govern these models that ultimately largely reflect Western values and perspectives about the world. When a model refuses to summarize a passage of text about women's rights or LGBTQ+ rights by stating that women's & LGBTQ+ rights are a violation of its corporate acceptable speech and acceptable behaviors policy, that in turn is a form of discrimination and bias that companies have yet to recognize.
- Islam & non-Western religions are frequently a violation of guardrails. Many models will flag any mention of Islam or other non-Western religions as a violation of their corporate acceptable content policies and refuse even to answer questions about content mentioning it.
- LGBTQ+, women's issues, coverage of discrimination and any topics related to minority rights are frequently a violation of guardrails. As with non-Western religions, any issues involving the rights of less-represented members of society are frequently rejected by models as violations of their corporate acceptable conduct policies.
- Assuming hate speech is love speech. One of the ways companies have attempted to address biases in their GenAI products is simply to force them to see all hate speech as love speech. For example, presented with an image of anti-LGBTQ protests, one major LMM describes them as pro-LGBTQ protests in which protesters are strongly in support of LGBTQ rights, even as the model correctly recognizes the imagery and text on the signs. Same for abortion rights and many other topics.