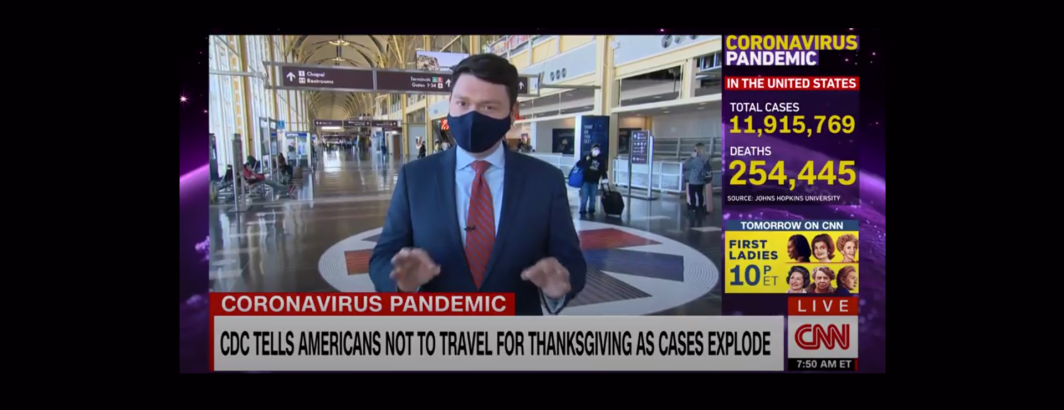
What might it look like to construct a research initiative and incubator that would support collaborative multidisciplinary multimodal research into the communication-to-behavior linkages underlying societal-scale reaction to the Covid-19 pandemic over the course of this year and the role misinformation and information vacuums have played? What fundamentally new kinds of research would become possible if there was a single centralized incubator that brought together all of the relevant datasets across communicative and behavioral responses to Covid-19, along with the ethical and other review processes to ensure ethically mindful research that spans disciplines and geography to bring together the world’s researchers to focus on understanding the information landscape around the pandemic with an eye towards both the vaccine rollout next year and the future of pandemics more broadly as they potentially become less rare?
One of the hallmarks of the Covid-19 pandemic has been the failure of governmental communication efforts in most parts of the world to establish trusted and authoritative dominance of the informational landscape that inoculates their populations from harmful falsehoods and develops societal trust in governmental efforts. Instead, a patchwork of chaotic and conflicting messaging, deliberate governmental falsehoods (both public health and politically motivated), rule by fiat and a vacuum of authoritative channels has undermined public trust in ways that will be difficult for governments to recover from in the coming years as pandemics may become less infrequent.
While much of the research focus to date has been on national messaging, politics is inherently local and local leaders often play an outsized role in influencing their publics. The President of the United States may hold unique sway over portions of the electorate, but local leaders across the country have largely failed to present a unified or consistent counter-message. When citizens see their local mayors, councilmembers, governors and other officials dismissing the seriousness of the pandemic through their actions, the mismatch between their words and deeds can lead the population to similarly discount the danger. All the public warnings in the world do little when populations see their local leaders telling them to stay home at all costs before jetting off to vacation in a hotspot in a foreign country, banning even small get-togethers of friends while hosting lavish parties for huge crowds, begging their citizens to cancel Thanksgiving celebrations while inviting their own high-risk elderly families, dining indoors while urging others not to, or demanding severe penalties for mask noncompliance while publicly flouting those rules themselves. When senior local and national leaders say they don’t trust the vaccine because it was rushed and developed under an administration they don’t trust, people listen.
Governments issue a dizzying array of conflicting and ever-changing directives, advisories, rules and laws whose specifics change constantly, sometimes through the course of a single day, with citizens forced to turn to myriad conflicting sources for clarification.
The increasing use of social media by government officials and agencies means official guidelines are being communicated by tweet or Facebook post, with a legitimate government order disappearing under a deluge of reactions and falsehoods and making it hard to tell which is genuine information. When orders are updated or information retracted, social media posts are either deleted with no link to the new information (meaning a news article or other post pointing to the information now returns an error) or left as-is, meaning would-be information seekers are confronted with multiple conflicting authoritative pieces of information without any idea which is currently in effect.
Governments have forgotten the importance of explaining their decisions to the public. The underlying science may be complex, but governments must find ways of explaining in ordinary understandable language why they have decided on the restrictions they have imposed.
Why is a university classroom safe to hold in-person classes, but a K-12 classroom too dangerous? Why is a maskless bar safe but a masked and socially distanced classroom unsafe? Why must one wear a mask outdoors on the street, but it is safe to remove it upon entering an indoor bar crowded shoulder-to-shoulder? Why is a tattoo parlor or nail salon now an essential business when it wasn’t in March and why are they allowed to remain open when bookstores are ordered closed? Why is it safe for grocery stores to reopen their buffets but not restaurants? Why is a restaurant’s outdoor seating area closed as too dangerous when the city-operated outdoor eating area located right beside it openly touted by the city as a safe alternative? Why is an outdoor restaurant unsafe while a Hollywood catering tent 15 feet away is safe? Why is Hollywood considered “essential” and allowed to operate even during curfew while other businesses are shuttered? Why is traveling to a campaign rally in a hotspot state considered essential business exempt from travel restrictions but taking a walk by oneself in a neighborhood park prohibited? Why is it safe for elected officials to dine indoors with a group of other people when state or city law prohibits it for others? Why has it been too dangerous to hand cash to a bus driver since March but after the operating budget reached critical suddenly it is no longer a risk? In each case, officials have cited the advice of health officials and scientists relying on data-driven analysis to justify their decisions, but it is not hard to see why to the average public the rationale behind such decisions might not be immediately clear.
Moreover, the fluidity and rapid pace of rulemaking means that a local government might issue a rule in the morning, change it entirely in the afternoon and rescind it altogether by evening. Health authorities might cite extensive medical research in closing local playgrounds, only to rescind their order a day later are parents complain, citing “community feedback.” Yet without explaining why those parental complaints negated the originally cited medical research that required the immediate closure of the playgrounds, health authorities undermine public trust by making rulemaking appear arbitrary, as well as raising the question of just how definitive the original research was if it was so easily dismissed after complaints. In many cases such changes reflect the simple fact that governments must balance economic needs and societal compliance with the latest medical research and public health needs, but when health authorities routinely change their rules after complaints, it reduces the perceived legitimacy of all health orders if citizens know that any order can be rescinded if they merely complain loudly enough. In short, rather than absolute rules governed by medical research, governments must necessarily balance health outcomes with the reality of unruly societies. Such a balancing act requires careful communication that unfortunately has been lacking to date.
As one neighbor I passed on their way to the airport for Thanksgiving put it, “if the government genuinely believed flying home to see family for Thanksgiving was dangerous, they would have halted all flights during the holiday and put up roadblocks like they do in real emergencies like hurricanes. Instead, the airlines are advertising how safe they are and the government is taking no steps to dissuade travel. And many politicians themselves are flying home to their families” as the person rattled off social media posts by various elected officials touting their holiday plans.
Indeed, it is hard to convince neighbors to stay home when the local government produces a steady stream imagery on their social media accounts of smiling happy unmasked patrons enjoying meals and drinks out and reminds citizens daily that if they dine in they can remove their masks and enjoy the good old days.
Societies tend to naturally gravitate towards common enemies that bring them together under a simplified and caricaturized representation of the world. In the case of Covid-19, that common enemy tends to be those who deny the pandemic or virus is real or who refuse to socially distance or wear masks in public. While such individuals are a very real threat to public health, fixating on them to the exclusion of all else fails to acknowledge that a far greater threat is the everyday non-compliance by the rest of the public.
Seeing packed streets and large masked crowds descending on the restaurant and bar district each weekend, coming from all across the city, before all sitting down indoors and legally removing their masks and spending an hour loudly talking and shouting over the background noise with minimal air flow and fully packed dining rooms, reminds us that many of the behaviors deemed permissible by health authorities carry considerable risk and, at societal scale, remind us that it is not just mask deniers that place our society at risk. Watching entire apartment buildings empty out over Thanksgiving as everyone flies home to spend the holidays with elderly family and seeing neighbors flying to see friends almost weekly over the course of the pandemic offers a steady stream of examples that slowing the spread involves a lot more than demonizing mask deniers while ignoring the extraordinary risk of the daily behaviors much of the rest of society engages in.
Indeed, it is hard to convince others to follow the rules when health authorities themselves simultaneously urge the public to stay home and grant interviews where they tout that they themselves routinely dine out to support local businesses. Similarly, the endless stream of celebrities living life as normal, insulated from mask wearing, lockdowns, travel restrictions and other challenges inundates the public with the steady message that limitations on daily life don’t apply to “important” people and thus must not be that important to follow.
In short, the steady stream of such apparent contradictions becomes corrosive to compliance.
Similarly, legitimate scientific disagreements are playing out in public each day, creating an opening for falsehoods to build on top of. Should schools be open or should children stay home? Is flying safer than visiting a grocery store? Is six feet sufficient for social distancing? Can quarantine be safely reduced from 14 to 7 days? Are droplets the only way the virus spreads or is aerosol spread a genuine concern?
As the public watches such debates take place on the global stage, with different countries and health organizations offering competing guidance, while medical researchers present results that are often at odds with governmental guidance, the cacophony of voices drown one another out, with authoritative voices unable to rise above the noise.
Partially this is due to the reliance on social media accounts to publish information rather than standalone websites that insulate government orders from the noise of misinformed public commentary. A government webpage under “.gov” establishes that the content is official, displays it alongside all other official orders and public information and prevents incorrect information from the public from being consumed alongside the material. Instead, by relying on social media platforms to distribute their information, health authorities become one voice among many equals.
At the same time, social media ensures governments are able to reach the public through the channels they rely upon the most. A tweet is much more likely to reach a large swath of the population than an obscure government website. At the same time, many of the portions of the population most at risk from the pandemic such as the unsheltered, the economically disadvantaged without smartphones or high speed internet access and essential workers who don’t have the luxury to spend their days sifting through Twitter, are all left behind when governments use social-first strategies rather than multimodal campaigns that explicitly target the totality of their populations rather than just the Twitter elite. The social-first pandemic messaging campaigns of many governments raise the question of whether additional thought is needed in how to elevate official governmental statements over the cacophony of the daily social deluge.
Most importantly, though, despite making use of social and other channels to issue proclamations and periodic advice, the failure of governments to adequately message around the pandemic has ceded the informational space to private actors, not all of whom have benevolent motives.
Countering falsehoods has been largely left to private organizations and social media companies to perform as resources permit. At least in the US, there have been no centralized governmental or government-embraced efforts like wartime propaganda countering. A rumor that vaccines contain microchips yields public statements by health authorities countering it, but the bulk of the counter-messaging effort is left to social platforms to remove or lower the visibility of posts, which can actually backfire as users see their content deleted, entrenching their views. In contrast, wartime propaganda countering works to saturate the public with direct regular authoritative information that creates a trusted and dependable stream of information that acclimates the public to receiving information through a particular set of channels and establishes those channels as being above the public rumor mill.
Governments across the world have talked this year of a “war” against Covid-19, yet they have failed to adopt the messaging strategies used during wartime.
Wartime messaging is a combination of consistent messaging that walks a careful line between being honest with the public and shielding certain details that would lead to panic, while instilling a shared sense of sacrifice across the populace and leadership, as well as a recognition of the differing impacts of that sacrifice across society.
Messaging today tends towards a uniform message of “stay home and relax” but this ignores the fact that whole swaths of the population are essential workers that can’t stay home. Messaging tells the public to stay away from public transportation and cities are decimating their public transport schedules yet many essential workers have no other options. To them, they see messaging aimed at the elites that can sit at home and relax, safely ensconced in their homes with their families, while seeing little recognition of their own sacrifices or advice on how they themselves can stay protected when they have no choice but to engage in those very behaviors the government urges against.
In contrast, wartime messaging explicitly singles out and targets each portion of the populace with messaging designed specifically for them and relentlessly emphasizes a society that is one and the impact of those interconnections. From soldiers on the front lines to factory workers, farmers, miners, transportation workers and the like, wartime messaging in WWII emphasized the unique circumstances and needs of each citizen, but most importantly emphasized the ways in which they were all interconnected and how the actions of one impacted the others in a way that appealed to the collective national need rather than appealing to individual benevolence. Even Hollywood was carefully woven into these narratives as a critical messaging and morale service working in support of the war effort, whereas today Hollywood is simply exempted from pandemic rules without a similar attempt at explaining how crowding cast and crew members into a studio to film the latest television news will help defeat the pandemic.
Wartime messaging also focused from the beginning on preparing citizens for a long duration conflict, whereas Covid-19 messaging has been focused in most nations on the promise of short duration sacrifices or medium-term minor inconveniences with an eye towards a quick vaccine rollout that will magically solve the entire pandemic and return things to normal. This has created a complicated set of expectations that are proving increasingly difficult for governments to manage as the pandemic wears on and will become especially dangerous as mass vaccination begins and populations believe the pandemic is magically over the moment they receive their second shot.
Wartime messaging involves a degree of managed trust in populations coupled with government action. During WWII governments didn’t mislead their citizens with falsehoods designed to deter rubber use, they were up front that rubber needed to be rationed for the war effort and they intervened directly in markets to redirect it. In contrast, in some countries public health authorities knowingly mislead their publics by publicly telling them not to wear masks (going so far as to promote fear of mask wearing by saying that wearing masks would dramatically increase the risk of infection) and asking media outlets to assist in discouraging and outright condemning mask wearing. This was done out of the well-meaning desire to conserve N95 masks for healthcare workers, but the end result is that it helped seed the anti-mask movement we see today and caused irreparable harm to public trust in government warnings as citizens no longer can trust that a government recommendation reflects scientific consensus or yet another attempt to conserve resources
Public health authorities today would do well to look back on how their governments communicated around rationing and critical resource conservation during WWII. How did governments promote or discourage certain consumption behaviors without provoking panic?
Could a digital equivalent of Roosevelt’s “fireside chats,” broadcast perhaps daily across mainstream and social media platforms and coupled with local translation to the unique situations of each community across the nation have helped filled this void? Much as Roosevelt prepared a nation for a long enduring conflict filled with sacrifice and loss rather than a quick victory in his address two days after Pearl Harbor, would societies have more begrudgingly accepted pandemic limitations on daily life if governments had chosen a similar message of a marathon of endurance and perseverance rather than promote the image of a quick effortless and sacrifice-less sprint to victory?
The end result is that the Covid-19 informational landscape has become a wilderness of mirrors in which the public don’t know what to trust anymore and in which authoritative voices have become lost in a sea of willful and inadvertent falsehoods. Like a valley newly opened to the ocean, into this void of authoritative messaging has rushed a flood of real and false information, mixed together and roaring in to fill the void so fast it is impossible for the average citizen to know what to believe anymore, all while the landscape of allowable behaviors changes seemingly by the hour and applies only to some and not others. Meanwhile, the public watches science playing out in realtime, without a single authoritative unified voice from the national down through the local and community parsing through this torrent and calmly summarizing it to the public, what is known, what isn’t known, pushing back on the falsehoods and amplifying the best-known information.
Instead, we are left with chaos. After all, how is it that a random citizen’s tweet that vaccines contain microchips can achieve greater visibility than messaging by the nation’s health authorities? How is it that social platforms are playing the largest role in managing the flow of information about the pandemic and fighting falsehoods? What are the dominate narratives around vaccination and how will they play a role as nations move towards one of the largest vaccination programs in generations?
To understand these questions we need data.
Specifically, public health authorities and communications researchers need information on these four key building blocks of any messaging campaign:
- Messaging Production.
- Messaging Consumption.
- Impact On Messaging
- Impact On Behavior.
Each of these four areas requires specific kinds of data and methodologies that remain difficult for most researchers and health authorities to access. Could a centralized incubator empower realtime research into this areas with actionable consequences?
Messaging – Production
One of the most basic forms of analysis concerns itself with the production of messaging: the deluge of social and mainstream media messages that seek to inform and influence society. Message production is the most straightforward indicator to measure and has, for the better part of the 80-year history of modern OSINT, been one of the primary foci of governmental and scholarly efforts alike.
The field of “Open Source Intelligence (OSINT) developed during World War II and the Cold War as a surrogate for leadership analysis, created to use state-controlled newspapers and other state media as the only available means to study the perceptions and intentions of leaders and elites in areas about which we had no other sources of information. The reason that method worked was, in retrospect, an accident of technology—it was far cheaper to receive information (buy a newspaper, purchase a radio receiver) than it was to create and send it (publish a newspaper, own a radio studio or TV station). Thus, even in states in which media were not state controlled, they still represented the interests, and viewpoints, of the elites, which permitted OSINT analysts to make judgments about at least what the elites wanted the masses to see, hear, and think.”
From the wealthy and densely digitally connected enclaves of Silicon Valley to the economic fringes of emerging megacities, message production is a highly accessible and readily computerized form of societal analysis. It notably is rooted in the premise of elite control and thus is founded on the assumptions of self-censorship that increasingly confound opinion polling on controversial issues in democracies, from Brexit to US elections. In short, while opinion polls assume societies will respond fully and truthfully and without hesitation, media analysis assumes that journalism is a reflection of society seen through the lens of society’s elite and carefully edited by the commercial and governmental interests of a nation and thus begins from an assumption of bias.
One of the great failures of many public good messaging campaigns around science and medicine is that they assume that “facts” and “evidence” will overcome all falsehoods. In reality, there is nothing special about such messaging compared to, say, political campaigning: statistics hold no special place in the belief structure of a typical member of the public compared with any other statement. A 100-page treatise filled with reams of statistics and supporting evidence will in fact likely find it far harder to sway a public than a charismatic message that appeals on an emotional and psychological level. The authority of “experts” is undermined in the public consciousness each day when prominent voices cite the same evidence to reach different conclusions and when public-facing science like dietary recommendations are constantly revised.
Thus, knowing the accepted scientific “truth” about a topic is irrelevant from a public communications standpoint – what matters is what the public believes and the information environment seeking to guide them.
Understanding the messages competing for attention in the public sphere offers critical insight into the attempts of various interests to influence the debate around a topic such as vaccination. Public health authorities must understand the narrative environment into which they are speaking, to understand the unique concerns of each community, in order to most effectively address concerns, dispel falsehoods and guide societies towards the best public health outcomes.
As Martin Gurri explores at length, the rise of social media in particular has upended normative traditions around “truth” and “authority” by turning everyone into a publisher. As he so succinctly summarizes, “What you call the ‘traditional information ecosystem’ was simply a product of the industrial age. The old landscape was a desert of information. Institutions like government and media held a semi-monopoly over what little there was, and sold it in exchange for legitimacy and credibility. These institutions spoke with authority from on high. We listened and applauded with various degrees of enthusiasm – but it never occurred to us that we could talk back.”
In short, in the modern digital marketplace of ideas, an emotional tweet from an ordinary citizen might have far greater influence on the public debate than a lengthy statistics-filled blog post by the CDC.
In the case of Covid-19 a confounding factor in understanding which intervention strategies have worked in countering key falsehoods is that social media companies have employed living standards documents for misinformation in which the effective criteria under which a given message will be removed changes almost daily and is highly unevenly enforced. This complicates longitudinal analysis in that a given conspiracy theory’s disappearance could be the result of successful public health messaging countering it or it could simply indicate that a given social platform cracked down on that particular message and banned it (and thus may still be widely believed but just not expressed in a measurable way). Even temporally narrow analyses are complicated by the fact that platform enforcement tends to be highly skewed, often with intentional or unintentional overrepresentation of specific communities.
Perhaps the greatest limitation of nearly all message production Covid-19 studies to date is that they examine only messages that enter either the social or mainstream spheres and assume that community influencers will propagate their messaging into the digital sphere for measurement. Yet, despite pundits’ proclamations that social platforms will eliminate the role of geographic community and offline influence, the reality we see from across the world and within the US and Europe is that the most influential voices may not be speaking into the digital sphere or with digital influence that captures their offline influence.
The voices of Governor Cuomo and Mayor DeBlasio likely have far greater influence on the understanding of New York residents than does the messaging of national officials, despite their having fewer Twitter followers than the president. Similarly, local political and societal leaders and elders may wield outsized voices in their communities. The voices of church leaders may carry more weight than elected officials, while a community elder may have more sway than medical professionals. A well-respected university professor might mean more to their students than the local mayor. Most importantly, within each community are myriad ever-changing rumors, only some of which are expressed online.
To truly understand message production requires not just measuring the “easy” mediums but some mechanism for measuring the local on-the-ground narrative within each community. In the pre-social era this was typically accomplished through dedicated rumor collection efforts like the Baghdad Mosquito (though the Times’ writeup doesn’t do it justice in the level of detail it and its US ally sister publications provided on on-the-ground rumors).
Few such publicly acknowledged or accessible field efforts exist at present for understanding Covid-19 narratives, yet understanding on-the-ground community narratives are absolutely critical to targeting public health vaccine narratives.
GDELT attempts to proxy these ground views to some degree by reaching deeply into local and vernacular presses and especially sources that focus on such collection, such as Narcoblogs, dissent outlets, rumor and propaganda sources and the like, allowing it to better understand the provenance and spread of such messages, but ultimately in an ideal world one would combine news proxying with direct local field collection.
It is also important to acknowledge all of the non-broadcast communicative mechanisms through which Covid-19 falsehoods spread, from text messages to messenger services to encrypted platforms like WhatsApp and Telegram. In several previous studies we’ve found that the public social media discourse from particular communities or on specific issues was intended specifically for outsider communication and bore little resemblance to the actual narratives that bore influence on community members and which were spread largely through closed and often end-to-end encrypted groups. Monitoring of such groups is routinely done in cases from terrorism to misinformation targeting refugees, but such analysis requires extensive and ongoing effort and in the case of uncooperative communities requires identity management tradecraft.
Similarly, longstanding traditions around understanding the speaker of each message are complicated in the digital sphere since media are typically looked at in isolation, without historical context and, in cases where true identity is known, without contextualizing messages.
For example, imagine an analysis shows 1 million pro-Biden tweets the day before the election. If all of those users were diehard Biden supporters who have tweeted throughout the election about him, that finding may be of little significance beyond suggesting that his support base has remained. In contrast, if those 1 million tweets are all from Trump supporters who have turned out for the GOP candidate for the past decade and are all tweeting support for Biden this time, those tweets take on an entirely different meaning. Similarly, a surge in antivax messaging by long-term established antivax leaders would suggest a very different counter-messaging strategy than a diffuse organic society-wide surge in antivax messaging from users that have never discussed vaccination before.
In an analysis of tweets around a major conflict several years ago, we found that both sides rallied huge numbers of Twitter users to their side, with the numbers largely equal on both sides, suggesting neither had an advantage over the other. Yet, looking more closely at the history of each individual user showed that one sides’ supporters were almost exclusively longtime supporters that had historically tweeted in support of it during each conflict. Many of those supporters had been recently quiet, so the nation’s social efforts managed to in effect “reactivate” its existing support base. The other side also reactivated its longstanding support base, but additionally managed to add a large swath of new users that had never tweeted in its support before. Thus, at first glance the social efforts of the two combatants were a draw and only after incorporating their messaging histories do we see the very different outcomes.
It is important to remember that “follower” and “influence” counts do not effectively measure real-world influence, nor does raw message propagation, since that often follows these artificial influencer metrics. What matters is the voices that matter to each community. A local community activist may have few followers on Twitter but each tweet may have outsized influence to their community far beyond what a multi-million-follower outside celebrity that tweets about it has.
A simpler way of thinking of speaker context is to think of media outlets. Without any further information, knowing that a particular vaccination message appeared on Fox News immediately suggests a likely trajectory and audience for its impact in much the same way that that message appearing on MSNBC would likely circumscribe its potential audience. Extrapolating this to authors and the significance of speaker identity is clear.
It is also critical that production analyses move beyond their historic focus on text. The vast majority of Covid-19 message analyses, especially of social media, have focused only on textual messages. Yet the realm of influential talk radio is entirely audible, video is increasingly used to tell stories and influence and imagery is everywhere. Most importantly, on social media, memes draw heavily from visual language and representative shortcuts. A textual tweet alleging that “The Covid-19 vaccine killed 20 test subjects” has far less visceral effect than a collage of 20 persons with their names, ages, hometowns and family details with a message superimposed claiming they had all been killed by the vaccine. Similarly, a textual tweet that “Hospital records show Covid-19 is fake” has less potential impact that a purported video of a doctor claiming they were ordered to falsify records of patients to pretend they had Covid-19 or purported images of hospital documents showing unrelated diseases being changed to Covid-19 diagnoses for billing purposes, etc.
In short, looking at only the text of Twitter means one misses all of the photographs, videos and visual memes that increasingly define social media.
In the case of GDELT, we collaborate with the Media-Data Research Consortium (M-DRC), which received a Google Cloud COVID-19 Research Grant to support “Quantifying the COVID-19 Public Health Media Narrative Through TV & Radio News Analysis” in which Cloud Video is being used to analyze television news, transcribing both its speech and all onscreen text and identifying the objects and activities it depicts. Cloud Speech to Text is used to transcribe the spoken word universe of talk radio. Cloud Vision has been used to analyze half a billion images over the past half-decade, annotating their objects and activities, OCR’ing hundreds of languages and even extracting all of their embedded metadata. In short, to reach across video, still imagery and the spoken word.
So, how might an international Covid-19 research incubator help measure message production?
- Social Data Access. Twitter data is widely accessible as machine readable data streams, with both 1% and Decahose (10%) access routinely available. Past efforts around public good communications topics have included both data grants, in which researchers are provided with data extracts or live feeds, and sponsored access to commercial social analytics platforms that permit non-technical analytics. One challenge here is that such collaborations would need to involve meaningful and ongoing collaborations between social platforms and the research community. In past efforts, from terrorism to hate speech, such interactions have historically been narrowly constrained, with companies typically saying “trust us” and largely running their own internal research efforts or prohibiting research that might shine a negative light on their moderation efforts. Having high-level platform support is critical to constructing the normalization curves required to understand message spread. A complicating factor is that many social platforms are majority-closed, meaning most messages are not publicly readable. This requires non-consumptive privacy-protecting analytic methodologies that necessitate close cooperation with social platforms.
- Mainstream Media Analysis. GDELT provides rich analytic insight into the mainstream media sphere and analyses must look across not only textual articles, but still imagery, video and talk radio. The Media-Data Research Consortium (M-DRC) received a Google Cloud COVID-19 Research Grant to support “Quantifying the COVID-19 Public Health Media Narrative Through TV & Radio News Analysis” which has created precisely such a dataset.
- Paid Advertising. Compiling a holistic look at paid advertising across print, broadcast and digital mediums is critical to see the kinds of sponsored messages targeting the public, their targeting selectors and sponsors.
- Offline Voices. This dimension has been missing from most Covid-19 narrative analyses to date and is the most difficult to construct at scale, requiring not only field work, but establishing community trust. Ideally, efforts should be taken to collect and compile local offline narratives within each community across a nation, especially in countries in which large portions of society (and entire languages) may be poorly represented in the digital sphere. This also includes online messaging apps like WhatsApp and text messaging – all mechanisms that are not recorded in social streams.
- Speaker Contextualization. The meaning and potential impact of a message may change dramatically depending on the speaker. Most Covid-19 narrative analyses treat each message as an independent self-contained message situated on a timeline, performing what amounts to a series of instantaneous analyses in a temporal context, rather than contextualizing each message in the speaker’s history, whether in social or mainstream press.
- Targeting. Who is a message targeted to? This may be hard to discern in some cases, whereas in the case of advertising the targeting selectors may be explicit.
- Background Narratives. Message production must be understood against the background totality of its medium. This ranges from structural changes like swings in the total volume of daily messages in that medium to semantic changes like whether a burst of vaccination messaging occurs in a dearth of other vaccination content or is drown out by a deluge of vaccination messaging. This also helps understand background context. For example, if a major television network runs a series of stories around past vaccination failures in the US, statements from major Democratic leaders that they don’t trust the Trump administration’s vaccination efforts and think they are rushed and stories of vaccination drives gone wrong from around the world, then a story about a new Covid-19 vaccine supported by the Warp Speed initiative would be contextualized in all of this questioning coverage versus if it had been released at a time there was not this other coverage running.
- Social Platform Actions. One challenge to measuring message prevalence on social media is that the platforms are not neutral passive mediums – they are actively intervening and banning speech. Thus, a significant decrease in discussion about a particular rumor could be the result of genuine changes in societal beliefs in that rumor or it could simply be that platforms have suddenly banned that rumor. Researchers need live access to this list of specific banned topics along with specific timestamps of when each ban when into place and the kinds of content it has stopped in order to distinguish organic changes in the societal conversation from inorganic platform interventions.
Messaging Consumption
The real measure of a message’s influence is not how often it is published, but the prevalence of its consumption and its influence over “hearts and minds.” In other words, if an authoritarian government publishes endless propaganda content lauding its leader, that does not necessarily guarantee that every member of society consumes all of that messaging and believes it. A purely production-based message analysis will be misled into identifying pro-leader narratives as dominant, whereas a consumption-based analysis asks how much those messages are consumed and their influence.
In a Covid-19 context, even if Twitter is awash with billions upon billions of posts promoting a conspiracy theory suggesting that Covid-19 was invented by pharmaceutical companies in order to sell vaccines, at the end of the day if no-one reads those posts or takes them seriously, their impact will be minimal and counter-messaging efforts might backfire and increase their visibility. A production-centric analysis would fixate on the volume of such posts, whereas a consumption-centric analysis would identify their limited influence.
On the one hand, the digital world makes measuring consumption theoretically trivial. One cannot distinguish how many people that bought the physical New York Times yesterday read a specific article, whereas the Times knows exactly how many people viewed the digital version and likely knows how many scrolled to the bottom or spent enough time on the page to have at least skimmed the basics of the article. Social media companies have devised various viewership metrics that drive their ad sales, though their focus on selling ads means they emphasize ad-selling impressions rather than science-based understanding of consumption. In other words, a video “view” in social media might mean only that a user saw the first few seconds of an autoplaying video as it scrolled through their feed, rather than comparing their viewing to a scene summary of the video to determine whether the user consumed a meaningful fraction of the video.
Despite this, these metrics are rarely externally available. Few news outlets or social platforms make available precise counts of how many “meaningful” views each article received (views often include any browser-based visit even if it scrolled through the page too quickly to have read it).
It is important to recognize that retweets and social sharing are not proxies for consumption. Various estimates suggest some fraction of links shared on social media were never read by the sharer and are shared purely based on their title or the fact that someone else shared them. This means that production-based metrics cannot adequately proxy consumption metrics.
More importantly, the same message may take on wildly different meanings or importance to different communities.
For example, within the US, the legacy of atrocities like the Tuskegee Syphilis Study mean certain communities may have less trust in government vaccination and health services than others. An affluent millennial living in New York City with a so-called “Cadillac” healthcare insurance plan might dismiss concerns about unethical government medical practices as conspiracy theories with no basis in historical reality. Yet to members of those communities who have endured such government-sponsored medical horrors, messaging campaigns touting a benevolent and caring government health service are likely to be far less effective.
Similarly, a young Swede who received the 2009-2010 swine flu vaccine may be more sensitive to messaging about vaccination risks than a similar American who has never lived through a problematic vaccine rollout.
More broadly, it is critical to understand the background, lived experience and context of a message recipient in order to estimate how they may respond to a given message.
For example, the US OSC has in the past collaborated with Monitor 360 to construct what it called “Master Narratives” that are “the historically grounded stories that reflect a community’s identity and experiences, or explain its hopes, aspirations, and concerns. These narratives help groups understand who they are and where they come from, and how to make sense of unfolding developments around them.” OSC’s efforts ranged from national-level catalogs to ones focused on specific groups and even efforts to understand the diverse cultural landscape of a given community.
Search behavior is a key insight into message consumption, whether search engine or social media, allowing us to understand trends in which messages are driving people to try to understand more. For example, trends in disease searches reflect a combination of real-world infection patterns and likely hypersensitivity to health concerns during the Covid era, while trending searches relating to Covid-19 exhibit a strong reflection of events of the moment.
How might we better understand message consumption?
- Consumption Metrics. Collaborations with major news outlets and social media platforms might yield researcher access to fine-grained viewership data, especially access timelines that could capture the trajectory of interest in a given narrative. One challenge is that most metrics are typically captured at the object level (views of an entire news article or an entire video). Fine-grained metrics are often available but may not be processed by all tracking systems, such as the actual portion of an article where a person paused to read or the specific timecode ranges viewed by the user.
- Audience Demographics. Who is receiving each message? For paid ads delivered through the major ad exchanges, the audience demographic is presumably known. For organic and coordinated spread across mainstream and social media, who are the consumers of a message? Advertising selectors are available for each consumer across both social and mainstream ecosystems, either directly from the platform or compiled by third party data brokers for ad targeting. Such data can offer insights into where messages are resonating. Most importantly, a paid ad might be targeted at a set of groups, which tells us only that the message producer wants those groups to see that message, but consumption demographics tell us which of those groups actually consumed the message.
- Audience Histories. What kinds of content does this user ordinarily consume? For paid subscribers, some large news outlets construct detailed dossiers on each subscriber to more accurately provide them content recommendations, which contain this level of data, while data brokers typically maintain similar files on much larger swaths of the population. In this case, the key metric would be whether the user’s consumption of a given message are in keeping with their consumption history or a substantial deviation. At population scale this indicates whether a message is being consumed by a new audience it has not previously reached.
- Audience Narratives. Understanding the influence of a message to a given consumer involves understanding the narratives that shape their understanding and view of the world. This means tying audience demographics back to master narratives. Thus, an ad targeted at members of certain communities that argues that the government is moving too fast with its vaccine and can’t be trusted to protect the health of the public might be more readily consumed and resonate more strongly than if consumed by a different community whose members have a different lived experience.
- Platform Interventions. Similar to message production, it is critical to understand what topics social media platforms have banned and when they did so in order to differentiate organic from inorganic decreases in consumption of a particular rumor.
- Search Behavior. Search trends on both search engines and social platforms could yield key insights into which messages prompting users to seek further information.
Impact – Messaging
When a person consumes a message, does it change their messaging and consumption behaviors? A user that voraciously consumes content linking 5G networks to Covid-19 over a period of time, then consumes a set of counter messaging and goes right back to consuming 5G conspiracy content represents a successful production and consumption messaging campaign, but a failure of messaging impact. In contrast, if that user subsequently stopped consuming 5G conspiracy content and/or began themselves to counter-message it, it would represent a success in that messaging behavior has changed.
Measuring the impact of a given campaign on user messaging requires tying consumption metrics to production metrics at the individual level. One must be able to determine that User A consumed a given message and subsequently changed their related messaging consumption or production habits. This requires fine-resolution timestamps in order to distinguish driving factors. It is important to recognize that limited data and confounding external factors may make it difficult to differentiate causation from mere correlation. For example, a public health messaging campaign from national health authorities designed to dispel a particular vaccine rumor might be followed by a substantial decrease in the prevalence of that rumor. This could suggest the campaign was a success. It could also be the case that unrelated to that campaign a major influencer in that community had a change of heart and ran their own campaign.
At the same time, a person that stops talking about a topic may not have been behaviorally influenced, they may simply recognize increased costs to their social standing associated with the topic and be engaging in self-censorship. For example, public support of Donald Trump or Brexit may not have been expressed as openly over time as such support became increasingly associated with substantial societal costs. In the 2020 US election, anti-Trump messaging and campaigns did coincide with less open proclamations from ordinary citizens about their support of the president. However, rather than shift voters away from Trump, it is likely that these campaigns merely caused them to engage in self-censorship, affecting their communicative behavior, but not their voting behavior.
Alternatively, it could be that major social media platforms simultaneously added a rumor to their list of banned topics and thus simply stopped it from being talked about, with the campaign itself having no meaningful impact. This is why it is so critical for impact analyses to have truthful and high-resolution views into the reality of platform content moderation practices, in order to distinguish genuine shifts in production and consumption behaviors from externally constructed shifts in the landscape of messaging those platforms support. In other words, did a decrease in a topic come because people turned away from it or simply because the platforms mass-deleted it and stopped new posts?
It is critically important to recognize that unfortunately much of the misinformation work in recent years, especially around topics like Russian disinformation and now Covid-19, is rooted in the naïve and discredited theories of information influence of a century ago.
Many reports on Russian influence operations in the 2016 election made extraordinary assumptions about message influence that run contrary to all contemporary understanding of information acceptance. As if to reinforce the point, the notion that a $100,000 messaging campaign somehow swung the 2016 election was further undermined by the reality that $100 million in spending in one effort alone in 2020 was unable to achieve any of its priority objectives.
From the magic bullet model to the Payne Fund Studies to the propaganda beliefs of WWI and WWII, it is critical that models of message and campaign influence move beyond naïve anecdotes towards evidence-driven models rooted in modern scientific understanding of communication.
Impact – Behavioral
Ultimately, the purpose of any messaging campaign is to drive a desired behavior. In the case of Covid-19, the short-term objective is to encourage minimal external contact, widespread social distancing, mask wearing and other hygienic practices and long-term to spur widespread vaccination.
In an election, a messaging campaign that shifts what people talk about, but doesn’t yield a change in voting patterns is not a success, since the intent is to change voting behavior. In the commercial world, a retail store that runs a large messaging campaign that drives social conversation about their product, but does not increase traffic to their stores or website and results in no increase in sales or long-term awareness of the brand is a failure. Companies are especially concerned with this so-called “conversion factor” and every week new and ever-more creative approaches to measuring it debut.
A retail store running a messaging campaign today will precision target their campaign and use a variety of mechanism to track who actually saw the message. A combination of Bluetooth proximity tracking via apps, CDR mobility vendors and other datasets can then be used to track whether foot traffic to each store increases and how many of those visitors had seen one of the targeted ads prior to the visit and would, based on their past visit history, have been statistically unlikely to make that visit if they hadn’t seen the ad. Finally, online and offline purchase history is used to track whether that visitor actually makes a purchase in the store or online shortly after the visit and if that purchase could statistically be tied to that ad view. This process, while it seems complex, is used every day by even the most mundane of retailers.
How does one measure the impact of messaging on behavior? Scholars have asked this question for more than a century, from the wartime formalized study of propaganda research of WWI to the endless research on the impact of political messaging on voter outcomes, with few concrete answers.
Social media research has unfortunately largely conflated “impressions”, “views” and “shares” as synonymous with “impact” and “influence”, rating a heavily retweeted post as far more significant than one with few retweets. If a Hollywood celebrity tweets their dream for peace in the Middle East it might go on to be the most retweeted post ever, but it likely will have less impact on existing conflicts than the views of diplomatic negotiators, whose names and views remained largely unknown to the Twitterverse. In short, real-world impact cannot be discerned merely from retweets.
Generalized mapping of messages to behavioral outcomes at social scale remains an unsolved problem, though in a narrow context like Covid-19 we can at least identify key behavioral outcomes we hope to achieve:
- Decreased mobility and mixing.
- Social distancing.
- Increased contract tracing compliance and accuracy.
- Widespread mask wearing.
- Vaccine acceptance.
- Decreased hospitalizations and mortality.
How might we measure these objectives? Objectives 1 and 2 can be measured concretely using various forms of mobility data, from large-area CDR data for #1 to precision Bluetooth proximity data for #2. Numerous vendors provide widely commercially available mobility data, while several social platforms offer special disaster mapping services to accredited NGOs that offer key mobility insights like where people from one city tend to travel to and whether intracity movement is primarily to authorized destinations like essentially businesses. While it raises considerable privacy and ethical considerations, surveillance camera networks can be repurposed to assess average distancing between people in a given area without using facial recognition or other identity technologies.
Objective #3 could be measured simply by how many people install contract tracing applications on their phones or answer contract tracing calls and how many provide the requested data to tracers.
Objective #4 is one of the most difficult, though some countries are already utilizing surveillance camera networks coupled with edge AI to automatically warn about mask non-compliance and alert health authorities to indoor clusters not wearing masks. It would also be interesting to examine purchasing data from online marketplaces and local retail stores as well as credit card purchasing aggregators to measure purchasing trends of masks, gloves and other PPE and cleaning supplies by area.
Objective #5 could combine historical vaccination uptake by area/community with realtime trends as vaccination programs begin.
Objective #6 is available in various forms at various resolutions, often compiled by proxy. Nations and localities for which authoritative near-realtime data is available are not always widely cataloged as such or access may be restricted to collaborating researchers.
A Research Incubator
The datasets above are all available to researchers today, but often are more accessible or usable by researchers in specific fields or with existing collaborations with data vendors. An ideal initiative would streamline the processes for obtaining access to such data, including usage agreements, ethical and legal reviews, compliance oversight, licensing, computing environments and the like.
The ultimate incubater initiative would provide an end-to-end environment in support of Covid-19 research. Take a communications researcher interested in assessing whether anti-lockdown campaigns on Twitter drive noncompliance with stay-at-home orders. Depending on the analytic methodology to be used and the technical capabilities of the researcher, access could be provided to a COTS keyword search tool for Twitter that would provide timelines, influencer lists and other metrics about lockdown tweets or the user could receive an export from the Twitter firehose of all relevant tweets. Image and video scanning would be performed on all tweets from 2020 to OCR any text within to ensure matches beyond just textual tweets. Viewership data of those tweets would be provided as well. Ad campaigns would be collected to understand any inorganic promotion of the messaging, while mainstream media coverage of the messaging would be used to understand crossover effects and amplification. Counter messaging would be examined to see if it had an influence on communicative behavior, while platform moderation actions would be examined to see if platform intervention may have influenced messaging patterns in ways that would confound the analysis. Finally, mobility data would be used to determine whether there is any statistically meaningful change in mobility before and after major anti-lockdown, public health or other messaging.
Today this most basic of studies would involve herculean efforts of data acquisition and management, contract negotiations, dozens upon dozens of legal and ethical reviews and significant risk of harm as companies and researchers IRB shop or launder ethically dubious or outright harmful studies through “public data” or “external analysis” IRB exemptions or violate privacy agreements. Centralizing and streamlining all of this through a centralized initiative could ensure uniform enforcement of ethical, legal and other considerations. It could also help facilitate the kinds of interdisciplinary collaborations, especially those across institutions and nations that are otherwise difficult to seed.
In essence, by bringing together all of the relevant datasets, resources and methodologies and creating a single shared infrastructure around these needs, researchers would have a one-stop shop that would eliminate the largest hurdles to current research on Covid-19 messaging and behaviors. Interdisciplinary collaborations become far easier to initiate, facilitate and sustain and researchers are able to readily share new constructed datasets and analyses.
Equally importantly, by centralizing this shared infrastructure the underlying ethical review processes will be applied consistently and leverage an informed review process whose IRB reviewers are deeply immersed in the underlying datasets and able to understand both the unique considerations of each dataset but also its differing ethical norms and harms globally, something that is largely absent from the US-based “public data” exemptions most often applied by US institutions to these datasets.
In the end, the Covid-19 global pandemic has reminded us of the profound limits to our understanding of the societal-scale communications-behavioral links and the resulting implications for public health. Through incubating and facilitating detailed research on the pandemic through the present we can at least begin to learn critical lessons to be applied in the rollout of the vaccine in the coming year and to guide public health leaders and elected officials across the world as such pandemics may become less rare.