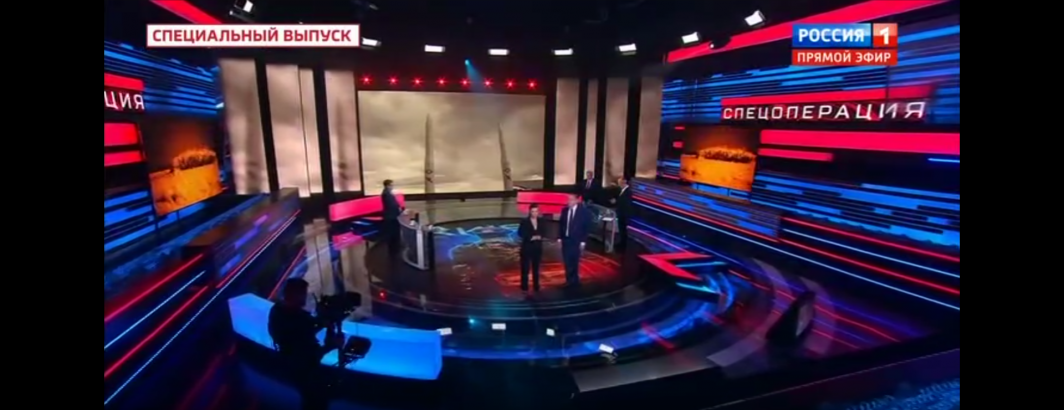
The past year has marked a watershed moment in the AI landscape, in which a range of applications have burst into the mainstream consciousness all at once, from human-like image, video and text generation and recognition to conversational AI. Each of these technologies crossed the threshold into real-world usefulness and most importantly, availability and accessibility to the general public, this year with a level of capability that profoundly upends our understanding of how AI may be deployed not in the future, but today. Yet, the real story that in its prioritization of public adoption and commercialization, the AI community has emphasized fluency over fidelity, designing systems that yield the kind of human-like output, the lack of which restrained the commercial potential of previous generations of these systems, without the corresponding systems to assess the fidelity and accuracy of those outputs.
For example, today it is trivial to build a toy machine translation model that yields human-like fluency so convincing that in real world use cases users often suspended their disbelief and embraced its results even when they contradicted their own knowledge of the language being translated. Most dangerously, efforts to debias and globalize AI have yet to rectify the immense imbalance between English and the rest of the world's languages. This results in models that generate grammatically flawless English that bears no resemblance to what was actually said in the other language. Even state-of-the-art production commercial systems that power our business world routinely mistranslate the most basic of factual data like "trillions" versus "billions" with immense impact on downstream processes, while research grade systems hallucinate entire stories, treating their source material not as text to be translated, but rather as a generative prompt to set off on writing their own fictional novella.
Even as the artificial benchmarks used by the research community tout immense accuracy gains in long-tail languages from the latest multilingual models based on vast new training datasets, when those models are deployed to real-world applications analyzing real-world data, they prove almost unusable beyond the same set of top languages the AI field has always emphasized. One recent state-of-the-art model optimized for similarity tasks and designed specifically to address long-tail languages and whose official benchmarks show it performing exceptionally well on Burmese fails trivially when applied to actual real-world Burmese text, scoring two near-identical Burmese articles as vastly less similar than two entirely disjoint English articles that bear no topical or grammatical similarity.
Most dangerously, the downstream communities for which these tools are designed are increasingly treating their fluency as evidence of fidelity and discarding their historic credulity – deploying the models based on a handful of small toy experiments without asking the most important questions about their accuracy at scale. When a speech translation model doesn't just skip over or repeat whole portions of a broadcast, but goes on to hallucinate its own unrelated stories, or, far worse, compellingly translate a passage so fluently it appears almost flawless, only to change the entire meaning of the text from delivering food to delivering natural gas to delivering weapons, each time it is run, it is time to step back and think more critically about the priorities of these new tools.
The AI community implicitly chases fluency in part because it has historically been the greatest obstacle to commercial adoption. A system that faithfully translates the meaning of a passage, but yields a stilted and disjointed translation "appears" less accurate to a consumer than a grammatically flawless translation that has nothing to do with the source passage but sounds convincing, in much the same way that a beautiful and intuitive user interface or visualization can make inaccurate data or a poor system appear more accurate and useful than a poor interface or visual.
What is needed most as we embark upon 2023 is a new approach to AI evaluation:
- Reporting Confidence. Models must be designed to place confidence front-and-center, passing through human-understandable translations of their inferencing process to yield not just explainable AI, but explainable AI that allows a downstream non-technical consumer of a model's output to understand how accurate the model believes its output is and to make an informed decision on whether to trust that output or not.
- More Accurately Measuring Confidence. For some architectures surfacing a model's confidence and adding it to the model's output is relatively straightforward. However, our own evaluations of some of the current approaches to confidence surfacing suggests that the current generation of models are so over-confident in their results that merely passing through this information as-is will not be sufficient – new approaches are required to allow models to more faithfully assess the accuracy of their outputs.
- Better Real-World Open Globalized Datasets. Many of the most profound advances in capability and accuracy over the past few years have come not from fundamental new algorithms, but rather simply from "emergent abilities" as training datasets cross certain thresholds of size and scale. Yet, these datasets still come from at-scale convenience crawls of the web, encoding its inherent biases and limitations. Urgently needed are more representative open, globalized real-world datasets for both training and testing. GDELT's unique "global local" emphasis makes it uniquely suited for such tasks and we are seeing exciting results from new collaborations in this space. Please reach out to us.
The coming future of AI could not be brighter as the underlying technologies, datasets, accessibility and applications have all crossed critical thresholds this year, but profound dangers lie ahead if the AI community continues to prioritize faithfulness over fidelity. Much as the promise of fully autonomous driverless cars has crashed head-on into the reality of its limitations and severely darkened the field's prospects, so too do the dangers of another AI winter increase so long as the AI community emphasizes adoption over accuracy.