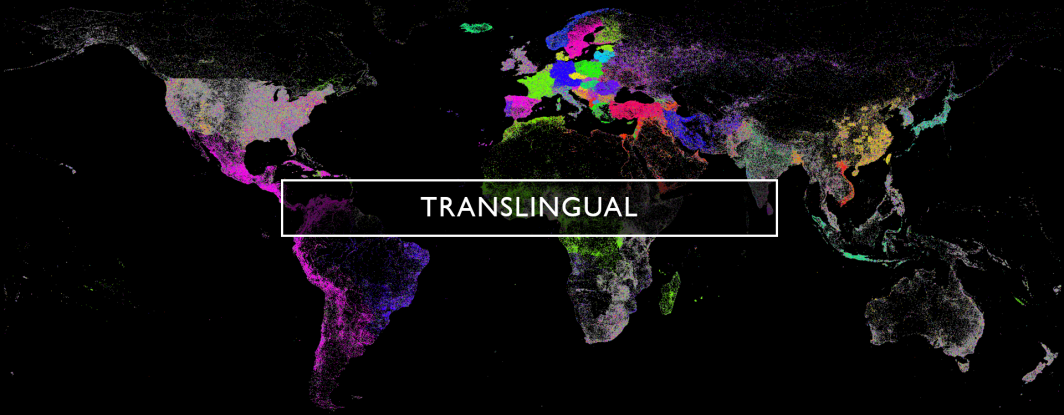
One of the most striking aspects of modern Neural Machine Translation (NMT) is the way in which even a small toy model, trained on just a small corpus, can yield human-like fluency in its production of target language prose. Even the smallest of models translating into a high-resource language like English can yield content that appears almost flawless at first glance. A closer inspection, however, reminds us that especially when translating from a low resource into a high resource language, the fluency is a result of the high resource target language, while the lack of training data in the source language can yield translations that are wildly inaccurate.
While BLEU and other scoring mechanisms are in theory designed to catch such imbalances and inaccuracies, the growing public exposure to machine translation and the greater visibility of fluency errors over translation errors means there has been an outsized emphasis on target language fluency prioritized over source language fidelity. In commercial systems in particular, target language fluency has become the highest priority, with modern SOTA systems restructuring the argument structure of the source text in its entirety, going far beyond grammatical and narrative normalization towards wholesale crafting of new prose out of whole cloth.
Such rewriting can be especially problematic when looking for specific phrasings or attempting to analyze contextual media framings, as a "radicalized terrorist on the national terrorism watchlist" becomes simply "a suspect" and a "mass casualty knife attack overwhelmed the ER, spilling into all available hallways, with many victims arriving deceased" becomes only "a few victims were treated for minor wounds," in which the translation entirely changes the meaning of the source text to the point that it no longer faithfully represents the meaning of the original material, despite having high English fluency.
As NMT systems place an ever-growing emphasis on fluency, it is critical that evaluations of such systems focus on scores that test their source material fidelity, rather than their target language fluency.