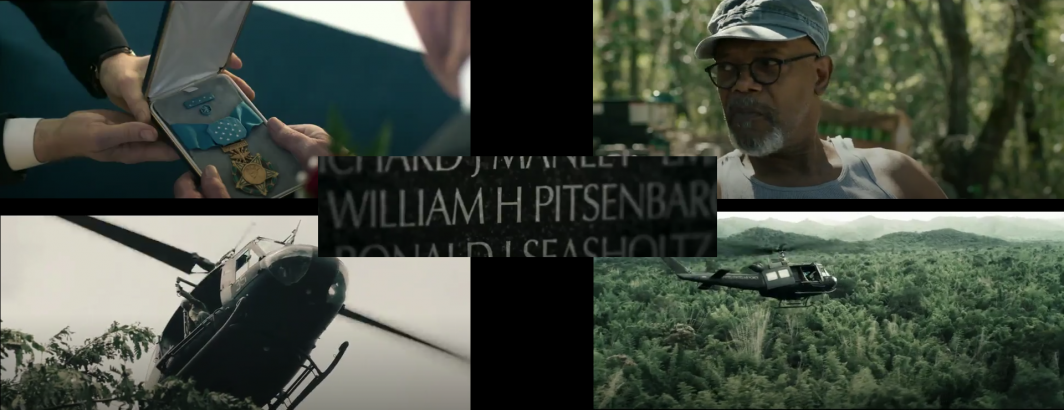
Machines watching television news record what they see with frame-level precision, using nanosecond timestamps. Humans performing content analysis on video typically record their annotations in seconds of airtime, using second-level timecodes when working at scale.
This creates unique challenges for reconciling automated precision with traditional human analysis. A single second of airtime can contain five entirely different scenes. What are the analytic workflows and research methodologies that allow us to look across these two very different content representations to understand the macro-level patterns of television news at scale?
The core Visual Global Entity Graph 2.0 dataset collapses the output of Google's Cloud Video API from its native frame-level resolution down to the seconds-of-airtime measure most common in traditional human-driven content analysis to make it easier for content analysts to get started. Today we released the underlying complete raw frame-level visual output of the Cloud Video API to enable researchers to compare these two representations and explore how this difference of temporal and spatial resolution influences the findings we can derive from television news.
We're tremendously excited to learn from what you find!