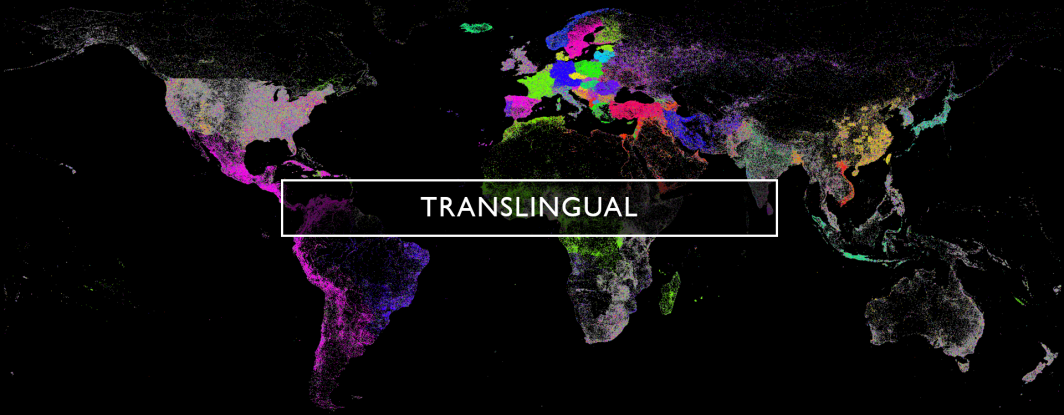
GDELT today spans more than 150 languages from across the world. With the release of the 150-language Web News NGrams 3.0 dataset this past December, it is now possible to perform at-scale truly multilingual linguistic analysis, training and testing that spans an unimaginable breadth of topics and linguistic use as diverse as the world itself. With GDELT 3.0's coming transition to its own inhouse 400-language detection infrastructure, its datasets will expand ever further into the world's rich linguistic diversity.
In contrast, much of computer science's focus on NLP and textual AI more generally has focused on English and a handful of major languages. While an increasing number of neural packages support a greater number of languages, their performance on languages beyond English and most common languages is typically far less, often abysmally so. As but one example, state-of-the-art research-grade benchmark embedding systems trained from the most exhaustive datasets available in the literature today will typically score two completely unrelated English news articles as being more similar than two nearly identical articles in a language like Burmese.
The dearth of available datasets beyond a handful of languages means that performance on tasks from entity extraction to topical extraction to Q&A are artificially inflated in the literature, with real-world performance dropping off a cliff when moving beyond English and the most common handful of languages. Dramatic skews in training datasets mean that even on relatively high-performing languages like Chinese, some tasks like translation will perform reasonably well, while others, like entity extraction, can perform extremely poorly. Worse, as language continues to evolve in realtime, training datasets typically lag by months to years to decades or are narrowly scoped to opportunistic collection or extremely misaligned or domain-constrained contexts, models age rapidly and real-world performance begins to decline almost from the moment they are deployed.
With GDELT's live realtime massively multilingual NGrams dataset, we are incredibly excited about the new opportunities available to NLP researchers in constructing new kinds of training and testing datasets that are updated in realtime to capture the live evolution of language and especially emergent terms and constructions like the wealth of novel and previously specialized terminology that emerged during the Covid-19 pandemic that confounded statically trained models. As GDELT continues to expand the number of languages it monitors beyond its historical 150-language threshold imposed by CLD2 to the 400 languages now supported by its inhouse language detection infrastructure, we are particularly excited for the opportunities to improve outcomes for long-tail language NLP development.
We'd love to hear from you with suggestions of local news outlets around the world we should add, especially those that expand our linguistic reach and we'd love to work more closely with the NLP and broader neural communities around the ways in which our immense open datasets can help move the field towards truly multilingual NLP and make AI more inclusive to the world's diverse languages.