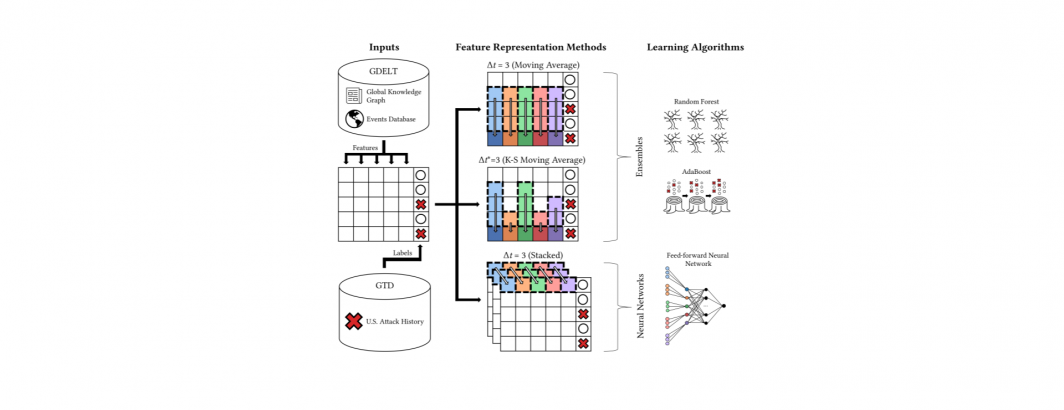
This study combines the GKG and EVENT datasets to incorporate thematic narrative underpinnings in the prediction of terror attacks:
Terrorism is a major problem worldwide, causing thousands of fatalities and billions of dollars in damage every year. Toward the end of better understanding and mitigating attacks that take place in the United States, we present a set of machine learning models that learn from localized news data in order to predict whether a terrorist attack will occur on a given calendar date and in a given state. The best model—a Random Forest that learns from a novel variable-length moving average representation of the feature space— achieves area under the receiver operating characteristic scores > .667 on four of the five states that were impacted most by terrorism between 2015 and 2018. Our key findings include that modeling terrorism as a set of independent events, rather than as a continuous process, is a fruitful approach—especially when the events are sparse and dissimilar. Additionally, our results highlight the need for localized models that account for differences between locations. From a machine learning perspective, we found that the Random Forest model outperformed several deep models on our multimodal, noisy, and imbalanced data set, thus demonstrating the efficacy of our feature representation method in such a context. Finally, we analyze factors that limit model performance, which include a noisy feature space and small amount of available data. These contributions provide an important foundation for the use of machine learning in efforts against terrorism in the United States and beyond.