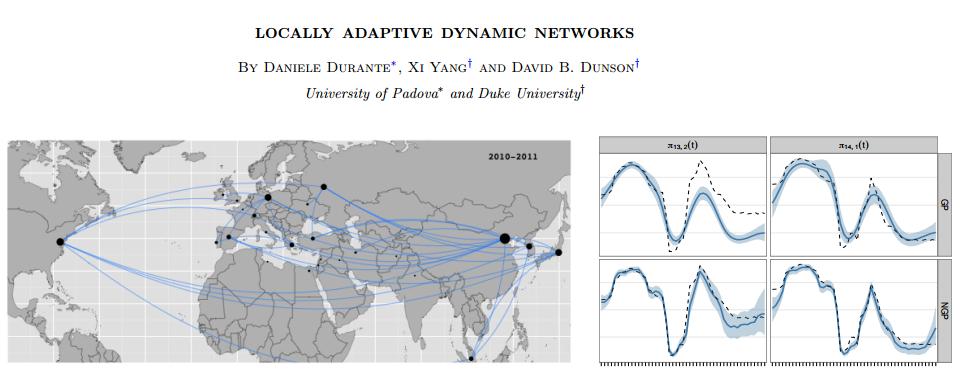
Researchers Daniele Durante (University of Padova), Xi Yang (Duke University), and David B. Dunson (Duke University) explore network inference using GDELT in "Locally Adaptive Dynamic Networks". The paper's abstract is:
Our focus is on realistically modeling dynamic networks of interrelationships among countries. Important aspects of such data that lead to problems with current methods include the tendency to move between periods of slow and rapid variation and the fine time scale at which data are available. Motivated by this application, we develop a novel methodology for Locally Adaptive DYnamic (LADY) network inference. The proposed LADY network model relies on a dynamic latent space representation in which each countries’ position evolves over time via a stochastic differential equation. Using a state space representation for these stochastic processes and Polya-gamma data augmentation, we develop an efficient MCMC algorithm and online updating procedures. We evaluate performance via simulation experiments, and consider an application to international relationships among countries during recent conflicts and financial crises.