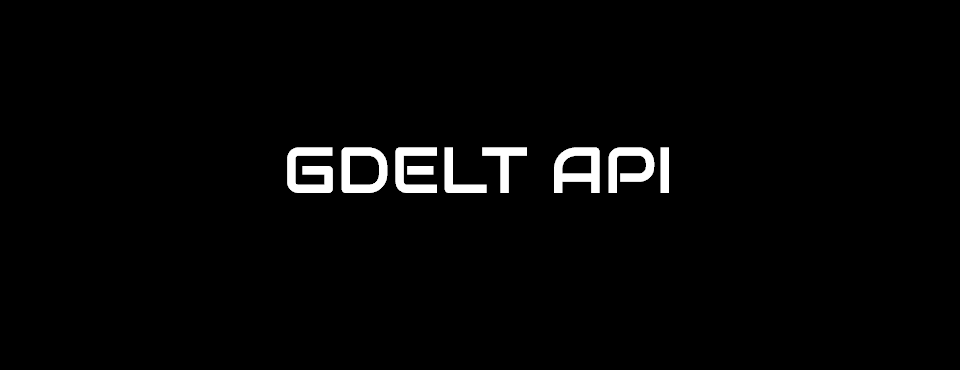
Historically the DOC 2.0 API has sorted its results according to the default ElasticSearch textual relevancy scoring model. Given GDELT's incredibly deep reach across the world, this means the API frequently returns as its top results coverage that is highly textually relevant but which originates from obscure small outlets that lack the broader focus and contextualization of coverage from international, national and regional outlets. To address this, after more than two years of research, a week ago we began experimentally incorporating additional signals, including the "popularity" of the outlet and its overall global "stature" among media outlets as a ranking signal that is blended with the article's textual relevancy score to yield its final ranking.
This is now the default ranking model for all searches of content published after 12:01AM September 16, 2018 – searches for content that include dates prior to this will utilize the original textual relevancy scoring model only. We will be constantly refining the underlying scoring models over time to yield the best possible results and once we have a final model that performs well in all scenarios we will retroactively apply it to our entire backfile and make it available for all searches. This mode is not currently available for image searches, only textual article searches.
Over time we will be greatly expanding the number of signals we incorporate into this ranking model, including localization signals, so the results of this relevancy model will continue to improve over time. One approach we've found great success with in past work has been to represent the entire media ecosystem as a single massive graph of news outlets and the propagation of discrete stories through that graph, assessing the aggregate trajectories of stories on particular topics through the graph from the moment the story broke to the moment it faded from coverage. We are also looking closely at the question of how to enable localization and customization of relevancy at the scale and diversity of GDELT's global user base. For example, a search for "impeachment" might be expected to bring up very different results for a user in the US compared with users in a number of other countries undergoing their own impeachment dramas at the moment. Addressing this at global scale is an incredibly complex topic that even some of the largest news search systems have yet to master. Along these lines we will be making a very exciting announcement next month regarding a massive new prototype system to allow you to create your own relevancy and summarization models that we hope will lead to entirely new research in this space, so stay tuned!
We'd love your feedback on the results you see and any suggestions of other metrics to incorporate into this model!