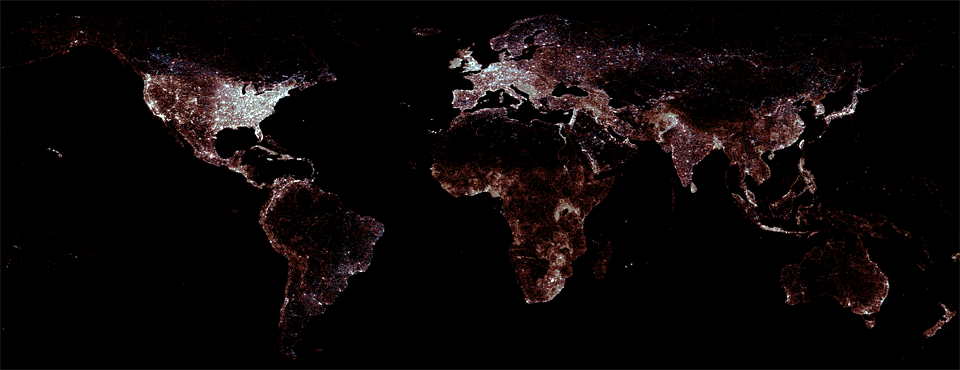
With the debut of GDELT 2.0, GDELT now monitors the world’s news media, including small local, citizen, and regional media outlets in the farthest corners of the earth, live-translating everything it monitors in 65 languages in realtime. Alongside all of that textual narrative, GDELT 2.0 brings to bear a portfolio of highly sophisticated algorithms to examine each article for potentially relevant images by examining their context with the article's narrative. This results in a list of images for each article that offers glimpses into the visual tapestry of the world's events.
One of the difficulties of operating at a truly global scale is that it isn't always possible to correctly identify whether an image is related to a given story based purely on its textual captioning or narrative context, and even the notion of "relevance" can differ substantially across applications. In the realm of disaster response, GDELT has been working closely with UN OCHA and MicroMappers to provide realtime information on sudden onset disasters around the world to their vast networks of volunteers to review and catalog. First deployed as a temporary pilot project for Typhoon Ruby this past December, the GDELT Live Disaster Stream became a production standing data stream earliest this month, and ended up seeing its inaugural deployment less than a week later in support of Cyclone Pam relief efforts.
A key lesson from the Cyclone Pam response has been the critical need to bring machine automation to the image triaging process. MicroMappers relies exclusively on human volunteers to determine both whether a given image is of relevance and to estimate the level of damage it shows and other characteristics requiring assessment of its visual content. Machine learning systems, while fairly accurate on textual material, have historically not had the level of accuracy or robustness to catalog the vast diversity of imagery found in disaster situations, from poor lighting and blurry focus to compression artifacts and poor quality. When cataloging the impact of a natural disaster, images of a local government official holding a press conference are likely of less interest than one of a convoy of aid trucks leaving the airport, which is itself of less interest than an image of a flattened village and its surrounding context.
To address this, we've been exploring the use of so-called "deep learning" approaches to image recognition, especially an emerging genre known as "convolutional neural networks" that have proven especially adept at offering robust high-quality image recognition even on the kind of poor-quality source material frequently found in disaster scenarios. One of the leaders in this emerging field is a startup called MetaMind and we've been particularly impressed with their technology and connected them with MicroMappers. Based on these early conversations and explorations, QCRI, MicroMappers, and MetaMind are now collaborating to apply MetaMind's deep learning tools to automatically filter all of the incoming imagery about a disaster both monitored by GDELT from global news media and submitted by volunteers or gleaned from social media, and filter all of that imagery into specific relevancy categories such that only imagery that has the highest likelihood of relevance is actually forwarded to the human volunteers.
When fully online, this will represent perhaps the most sophisticated assessment pipeline ever created for estimating the damage and impacts of a natural disaster in realtime. All global news coverage monitored by GDELT from the most remote regions of the earth, live-translated from 65 languages, will be scanned for the latest list of active disasters from OCHA, and all potentially relevant imagery from that coverage will be examined by MetaMind's deep learning algorithms to determine whether its an image of a food convoy or a photograph of a flattened village, with the images ultimately dispatched to a global network of human volunteers for final assessment, creating a heavily automated global-scale image assessment pipeline for global disasters.