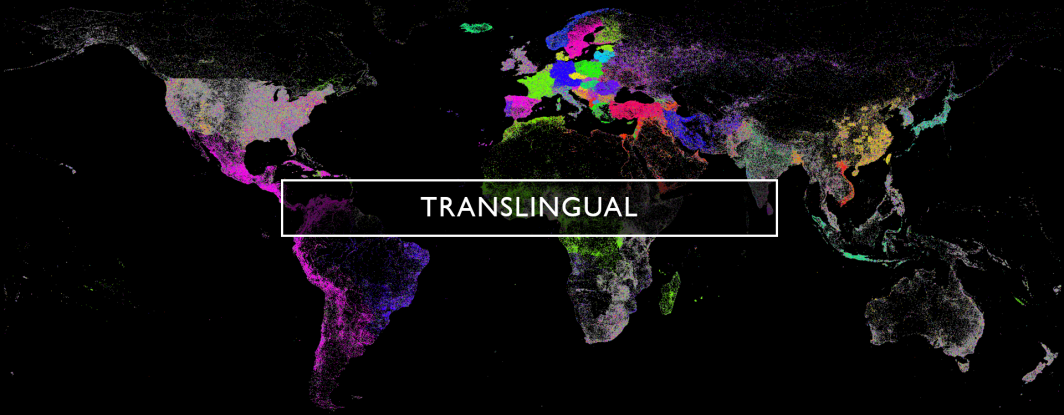
As we prepare the second generation of our massive GDELT Translingual global machine translation infrastructure, we are leveraging the wealth of insights and lessons learned we have gathered from Translingual 1.0.
One area we are focusing substantial effort on in the new generation of Translingual is the importance of nounphrase recovery and the tension between grammatical fluency, fidelity and faithfulness and the impacts of these driving forces on the recovery of nounphrases across languages. Given GDELT's vast linguistic archives spanning the 152 languages we monitor across every imaginable topic over the years, we have been working closely on understanding the real world ways in which concepts and names are expressed across each language. In particular, the pandemic's sudden and rapid spread of myriad novel, repurposed and specialty words has reinforced the need for translation systems to be able to rapidly evolve their understanding of language use.
Most importantly, however, we have seen that to maximize their ability to faithfully recover nounphrases, translation models must understand them separately from their understanding of language more broadly, treating them not as words and phrases on a page, but rather as underlying high-order concepts or entities. Over the coming weeks we'll be making some tremendously exciting announcements about Translingual 2.0's new approaches to translating the world's news at planetary scale!