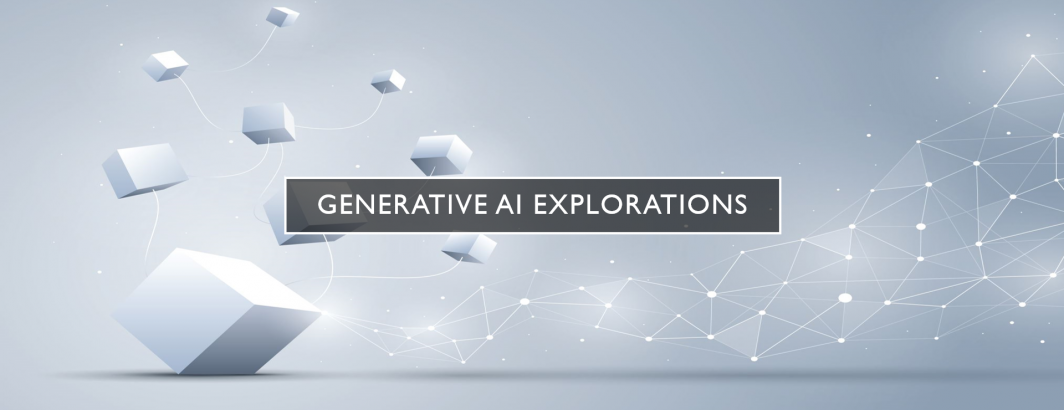
Yesterday we demonstrated how "generative search" can actually become "plagiarism search" in which, rather than summarize search results, LLM copy-pastes those results and/or its training data verbatim and presents them as its own work. As companies increasingly explore the summarization and distillation capabilities of LLMs, many of the enterprise applications being discussed are prefaced on an assumption of originality: that rather than copy its source material verbatim, LLMs actually synthesize and express it in their own words.
For example, a commercial abstracting service that uses an LLM to summarize academic articles and sells its machine-generated summaries as a service would face significant potential legal exposure if it turned out that the abstracts it sells were largely composed of verbatim copy-pasted text from the source material instead of novel text conveying a distilled version of its meaning. Even a company that performs such abstracting solely for its own internal use and distributes the resulting summaries only to its own employees would likely face similar legal risk if the LLM-generated summaries inadvertently simply draw from the source material instead of writing their own content.
Most production LLMs allow adjustment of the inference "temperature" as a way of effectively controlling the level of "creativity" they exhibit. Increasing temperature likely decreases the risk of verbatim copying, but increases the risk of hallucination and other adverse effects and thus companies tend to utilize relatively low temperature settings to encourage stricter adherence to the source material. This creates an inherent tension between the risk of plagiarism and the risk of hallucination.
Companies deploying LLM-based text generation should perform in-depth experimentation on their LLM solution prior to deployment to assess the degree to which it passes through either training data or source text (or both) and implement output monitoring that automatically aborts output if too many of the underlying clauses are found in either dataset, suggesting content plagiarism.