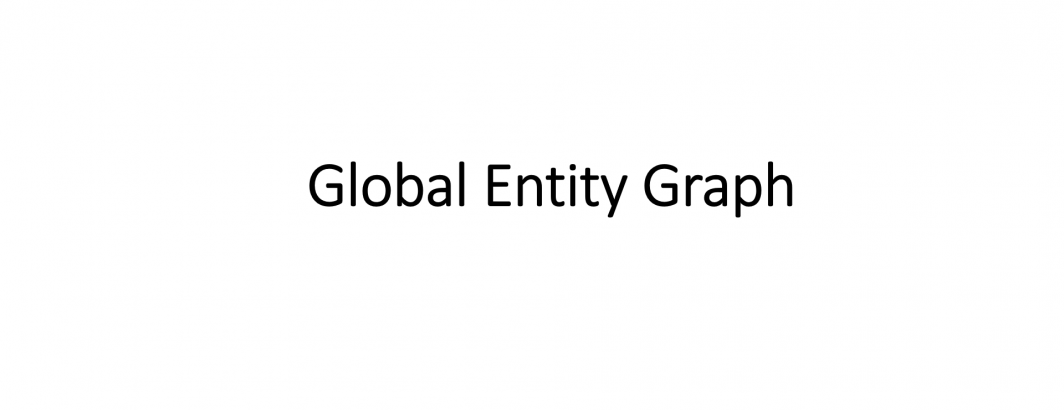
The inaugural GDELT Global Entity Graph (GEG) contained more than 11.4 billion entity annotations from over 103 million English language articles 2016-2019, annotated through Google's Cloud Natural Language API. What are the most common entities found in global English language online news coverage of the past three years?
Out of the 11,416,958,078 total entity annotations, a total of 1,584,550,381 entities (13.9%) have a non-null MID. Of the entities with a MID code, there were 27,833,754 distinct entity names resolving to 13,892,261 distinct MID entity IDs (name variants of an entity all resolve to the same MID).
The entities with MID codes fall into eight "types" as seen below:
Type | Count |
PERSON | 6332329 |
LOCATION | 2849856 |
ORGANIZATION | 2196649 |
OTHER | 1326627 |
WORK_OF_ART | 795123 |
EVENT | 253562 |
CONSUMER_GOOD | 136813 |
UNKNOWN | 1302 |
For each entity, its most common name, MID, most common type, most common Wikipedia URL, average salience and total number of appearances was computed. Since the Cloud Natural Language API recognizes many different alternative and variant names for entities, the 11.4 billion entities were collapsed by MID code (entities without a MID code were excluded). For each MID code, a list of every textual name associated with that MID was compiled and the most common one selected, with the same process applied to its type (since a name in different contexts can take on different type roles) and Wikipedia URL. The average salience of all mentions of the entity across the 103 million articles was also recorded, allowing entities to be examined through how "important" they are on average to the documents they appear in, while the total count reflects how often the entity appeared.
The top 10 entities appear below:
Entity | MID | Type | WikipediaURL | AvgSalience | Count |
U.S. | /m/09c7w0 | LOCATION | https://en.wikipedia.org/wiki/United_States | 0.014269125 | 31447173 |
Donald Trump | /m/0cqt90 | PERSON | https://en.wikipedia.org/wiki/Donald_Trump | 0.102800737 | 12805048 |
UK | /m/07ssc | LOCATION | https://en.wikipedia.org/wiki/United_Kingdom | 0.010535596 | 8679941 |
The Associated Press | /m/0cv_2 | ORGANIZATION | https://en.wikipedia.org/wiki/Associated_Press | 0.012310849 | 7978374 |
Europe | /m/02j9z | LOCATION | https://en.wikipedia.org/wiki/Europe | 0.005559554 | 7367460 |
New York | /m/02_286 | LOCATION | https://en.wikipedia.org/wiki/New_York_City | 0.006931887 | 6991149 |
Republican | /m/07wbk | ORGANIZATION | https://en.wikipedia.org/wiki/Republican_Party_(United_States) | 0.010974562 | 6672985 |
/m/0289n8t | OTHER | https://en.wikipedia.org/wiki/Twitter | 0.004768608 | 6662767 | |
China | /m/0d05w3 | LOCATION | https://en.wikipedia.org/wiki/China | 0.024354636 | 6460596 |
Democratic | /m/0d075m | PERSON | https://en.wikipedia.org/wiki/Democratic_Party_(United_States) | 0.011383625 | 6126569 |
The complete list of 13,892,261 distinct MIDs is available as a UTF8 CSV file:
- Master List Of MIDs In GEG-GCNLAPI 2016-2019. (346MB compressed / 937MB uncompressed).
TECHNICAL DETAILS
Compiling the dataset above took just a single SQL query and 153 seconds to process 382GB of annotations into the final dataset.
SELECT APPROX_TOP_COUNT(entities.name, 1)[OFFSET(0)].value entity, entities.mid mid, APPROX_TOP_COUNT(entities.type, 1)[OFFSET(0)].value type, APPROX_TOP_COUNT(entities.wikipediaUrl, 1)[OFFSET(0)].value wikipediaurl, avg(avgSalience) avgsalience, count(1) count FROM `gdelt-bq.gdeltv2.geg_gcnlapi`, unnest(entities) entities where entities.mid is not null group by entities.mid order by Count desc