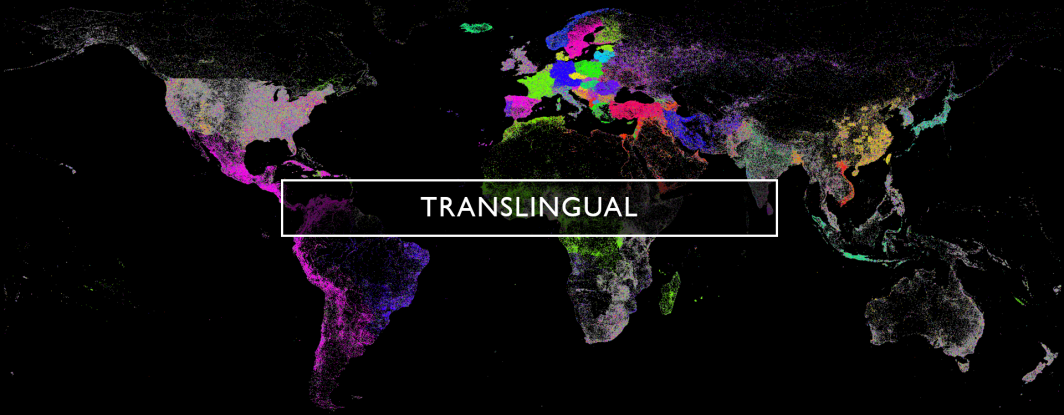
GDELT has long relied on CLD2 in "extended mode" to determine the language of each article it crawls, allowing it to detect 167 languages. (CLD2's default mode detects 80 languages, while its extended mode provides both detection and mean confidence thresholding scores for 167 languages (not all languages offer scores), excluding artificial languages like Klingon and very rare languages which raise the total to a potential of 209 languages). While extraordinarily fast and high accuracy for the most common languages GDELT encounters, CLD2's accuracy degrades substantially on less common languages. It is also not readily extensible beyond its 167 extended languages, limiting GDELT's ability to continue to reach across the ever-growing linguistic diversity GDELT is tasked with understanding.
Despite these limitations and despite the enormous advances that have occurred in the subsequent decade since its release, language detection has not advanced as far beyond CLD2's capabilities as other areas of NLP have since that period. CLD2's ubiquity and simplicity means that many newer language detection toolkits actually rely on it in whole or in part. Newer neural approaches to language modeling like fastText's language detection are still typically limited to the same set of languages, typically around 160 to 180 languages, with substantially degraded accuracy at the tail end, especially for languages which share some degree of linguistic structuring and lack their own distinguishing characterset distinctions. Despite the availability of vast new archives of training data today, the performance of even state of the art neural language modeling tools degrades so sharply on even reasonably accessible low-resource languages like Burmese that their utility for real-world use is seriously challenged.
At the same time, as we start to look beyond today's world of computational linguistics focusing on a small number of languages towards a future world in which every language is equally represented in our computational pipelines, we recognize that one of the first steps in that process is more accurate language detection that covers more of the world's rich linguistic diversity.
Towards this end we are exploring a number of approaches that leverage our vast reach into local news around the world and hope to have more to share soon!