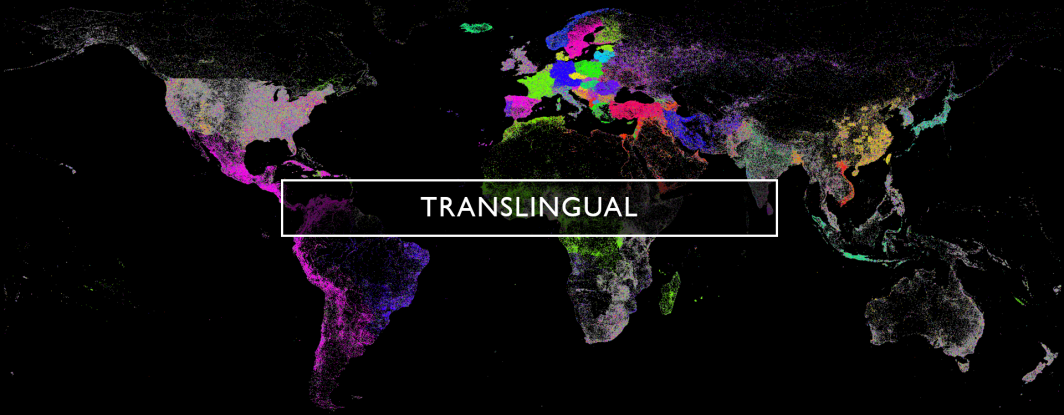
One of the applications we are most excited about with our new many-to-many translation graphs in Translingual 2.0 is the ability to combine multiple monolingual toolkits into a seamlessly bilingual analysis pipeline. For example, an English-only geocoding system could be applied to the English translation of an Arabic news article, identifying all of the geographic locations it references. Using the translation graph, the English offsets of each geographic reference could be crosswalked into the equivalent offset(s) in the Arabic text (since multiple words at different sentential locations in one language may combine into a single word in another language) and used with an Arabic-only entity-level sentiment tool to compute the sentiment of each location mention.
Bilingual KWIC ngram datasets in which we compute contextual keywords in both the original and English translations for a given entity or topic will open the door to a range of new NLP applications that we hope will seed new interest and research into native tools for the world's languages beyond those that have typically received the most attention. In particular, using English as a universal intermediate language, it becomes possible for example to look across the world's languages for references to climate change and then compute snippets in the original languages for language-specific NLP research, allowing rapid and trivial multilingual KWIC construction in ways never even imaginable before.
We are working on a number of approaches to making these graphs searchable and analyzable through the new GDELT Analysis Service that will launch towards the end of this year and we are immensely excited by the potential of this new architecture to open the door to much greater crosslingual research.