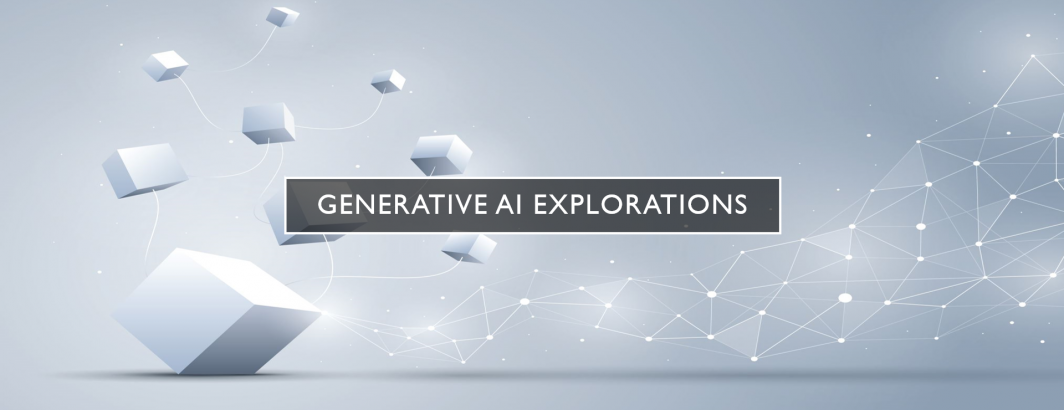
For more than six decades digital search has been based on the humble keyword. A search of a document database for articles about a "CEO" required only that the article contain the literal word "CEO" (perhaps capitalized or not) or stemmed or boolean-based variants (depending on the query) and returned the articles that matched, typically ranked by some form of TFIDF or similar frequency-based scoring. A 500-word article about a white male CEO that mentioned the word "CEO" 7 times in the text had just the same likelihood of being returned as the top result as a 500-word article about an African American female CEO that mentioned CEO 7 times in the text. Gender, race, background and the myriad other characteristics of being did not factor into keyword searches.
In contrast, our new world of semantic and generative search are based on embedding models that have been trained on vast web-scale archives of content to learn the similarities in how words are used. They encode that a "doctor" appears more often with "nurse" and "medical professional" than "concrete" and that "dog" and "canine" and "golden retriever" are all used in highly similar contexts. In this way, a search for "doctor" can return an article that mentions only "medical professionals", while a search for "dog" can return a profile on "golden retrievers" and so on.
While these are immensely powerful capabilities that allow searches to abstract beyond literal word choice, they present profoundly difficult challenges: how to address the innate societal biases encoded in these models? At the end of the day, a keyword search for "ceo AND leadership AND success" represents a conscious choice by the searcher of how to define their conception of a CEO. Importantly, different searchers can use different definitions simply by adjusting their search terms. In contrast, embeddings enforce the same definition of each term on all searchers and learn their definitions from their training data. A model that was trained largely on historical data that largely described male doctors would encode that a search for "doctor" should return only articles that also contain male pronouns and display those with female pronouns only if there is any room left on the results page.
This is why today when using at least one major commercial embedding model that is widely touted for semantic search applications, searches for "CEO" will return profiles of white men first, then African American men, then at the bottom an even mixture of female CEOs.
Companies today have become so used to a world of exact match keyword search that it is not even on their radar that embedding-based semantic search might impose existential gender and racial biases on their search results. A company that uses an embedding-based semantic search engine will suddenly impose myriad hidden biases on its users' searches without even realizing it. A news media organization that replaces its keyword-based search engine with an embedding-driven semantic search system will likely significantly bias its results towards articles featuring certain genders and races. In our world of traffic-driven digital newsrooms, that might, in turn, spur editors to assign more articles featuring those people due to their traffic-driving nature and deemphasize others who are simply not being searched for, without realizing that all of this is the result of those biased models.
Worse, even companies that have dedicated focal teams on bias and trust often wrongly assume that modern LLM-based embedding models follow in the footsteps of the massive focus on embedding debiasing of the past decade in which greater attention to biases like "doctor => man, nurse => woman" led to heavy investments in new training and debiasing practices. In reality, LLM embedding models have wiped the slate clean in that regard, eliminating all of the debiasing work of recent years and restoring all of those innate biases back to their full strength.
In the end, companies must recognize that the brave new world of semantic and generative search represents an existential change from the neutral past of exact match keyword search. Search today encodes myriad unpredictable biases that existentially shape their search results, creating legal and ethical challenges that companies will have to address as societies become more aware of these invisible shaping hands.