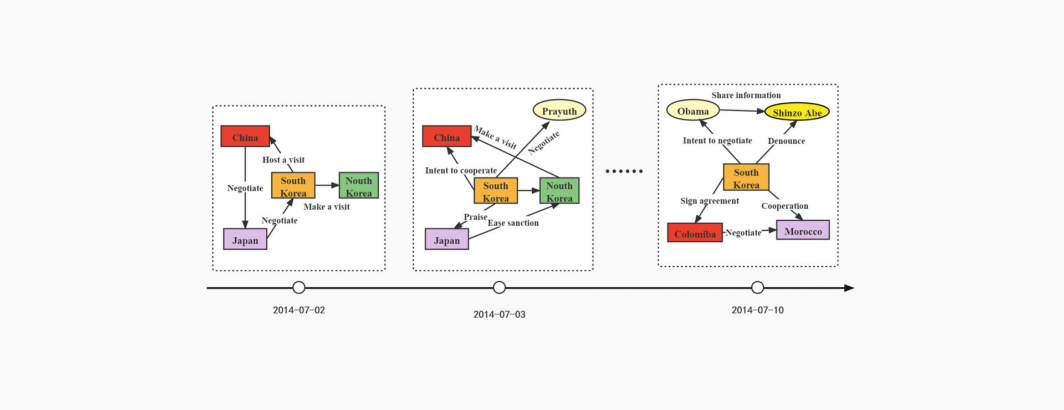
The research on temporal knowledge graphs (TKGs) has received increasing attention. Since knowledge graphs are always incomplete, knowledge reasoning problems are crucial. However, knowledge reasoning is challenging due to the temporal evolution of TKGs. Yet, most existing approaches focus on knowledge graph inference within past timestamps and cannot predict upcoming facts. There is evidence that when temporal facts in TKGs are evolving and interacting, most of them exhibit repeating patterns along the historical timeline. This observation indicates that forecasting models may predict upcoming facts based on history. To this end, this paper proposes a novel temporal representation learning model for predicting future facts named DAuCNet, which applies a Deep Autoregressive structure as the main framework and combines it with a time-aware Copy-based mechanism Network. Specifically, our model proposes a Temporal Fact Encoder to encode historical facts and a Duplicate Fact Collector to collect historically relevant events and identify repetitive events. It employs a multi-relation Neighborhood Aggregator based on graph-attention networks to model the connection of facts at the concurrent window. Finally, DAuCNet integrates these three modules to forecast future facts. Experimental results show that DAuCNet performs significantly better at temporal link prediction and inference for future timestamps than other baselines using five public knowledge graph datasets.