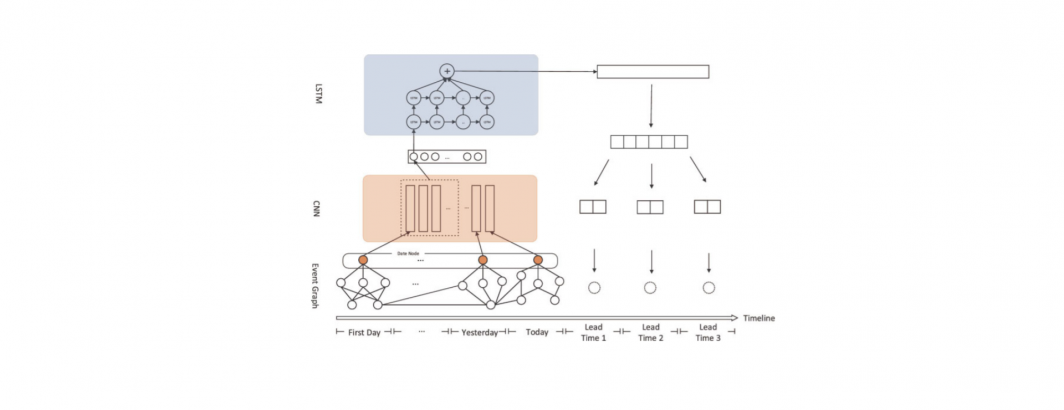
This paper by researchers at the University of Electronic Science and Technology in China presents an event forecasting framework:
Having the ability to forecast civil unrest events, such as violent protests, is crucial because they can lead to severe violent conflict and social instabilities. Civil unrests are comprehensive consequences of multiple factors, which could be related to political, economic, cultural, and other types of historical events. Therefore, people naturally organize such historical data into time-series data and feed it into an RNN-like model to perform the forecasting. However, how to encode discrete historical information into a unified vector space is very important. Different events may have extensive and complex relationships in time, space, and participants. Traditional methods, such as collecting indicators of various fields as features, miss the vital correlation information between events. In this work, we propose a Graph Neural Network based model to learn the representation of correlated historical event information. By using the dates, events, participants, and locations as nodes, we construct an event graph so that the relationship between events can be expressed unambiguously. We organize date-node’s representations into time-series data and use an LSTM to predict if there will be a violent protest or demonstration in the next few days. In the experiments, we use historical events from Hong Kong to evaluate our system’s forecasting ability in 1-day, 2-day, and 3-day lead-time. Our system achieves recall rates of 0.85, 0.86, 0.88, and precision rates of 0.75, 0.77, 0.75, respectively. We also discussed the impact of longer prediction lead times, and external events in China Mainland, the United States, and the United Kingdom on the Hong Kong civil unrest event prediction.