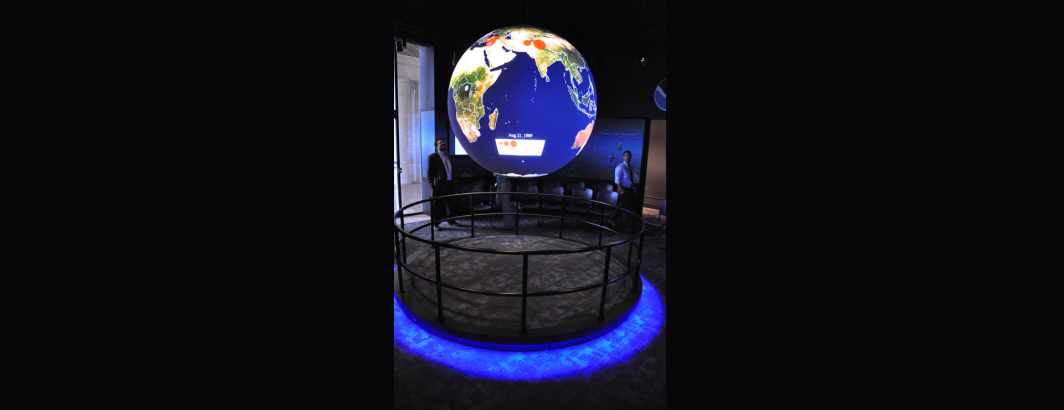
It is remarkable that just over a year and a quarter ago, on Dec. 31, 2019, BlueDot sent one of the very first alerts warning of the impending Covid-19 pandemic using data GDELT monitored the previous day. Its machine learning algorithms leverage GDELT's vast reach into local coverage in local languages around the world, scanning the GDELT GKG in realtime to identify the earliest glimmers of potential disease outbreaks and coupling those alerts with overlays like transportation networks, mobility corridors, health infrastructure and other information to estimate disease trajectories.
Even more remarkable is that despite GDELT having no explicit focus on biosurveillance (it covers all topics), these early warning signals appeared in GDELT's open data feeds at the same time that some of the world's largest dedicated biosurveillance monitors saw their first warning signals. In short, for all the talk about social media monitoring "beating the news" and the predictive power of dedicated purpose-built disease monitoring systems, GDELT's extraordinary reach across local media in local languages across the world caught the earliest glimmers of Covid-19 at the same time as these other systems and long before the pandemic began trending on Twitter. Indeed, the same held true for the 2014 Ebola outbreak, in which GDELT monitored the first glimmers of the outbreak before these other platforms, leading to the creation of our mass machine translation infrastructure to expand this early warning capability.
Today we are working on expanding this capability to not just provide the earliest warning signals of impending outbreaks, but to improve how governments and health authorities communicate with the public, engage with public narratives and combat falsehoods during public health emergencies by offering ever-richer understandings and modeling approaches for codifying public health narratives.
As we look to the future, it is worth reflecting back on this 2016 vision we proposed to US public health authorities to create "A Realtime Global Biosurveillance Platform for Mapping, Forecasting, Network Assessment and Community Outreach for Infectious Disease." Unfortunately, our proposed biosurveillance platform was ultimately dismissed in favor of legacy tools and Twitter scraping, with reviewers dismissive that mass multilingual news monitoring and translation of local media across the planet could offer early warning signs of disease outbreaks, the importance of translation of local language local sources or that a platform outside of the medical community could be used to identify outbreaks. Ironically, GDELT's early warning signals of Covid-19, flagged by BlueDot's machine learning algorithms and which lead to their worldwide alert, actually beat or equaled those same dedicated massive and human-intensive biosurveillance efforts touted at the time by reviewers, showcasing the power of global-scale all-topics worldwide monitoring of local media coupled with machine translation and sophisticated analytics.
You can read below what might have been.
A Realtime Global Biosurveillance Platform for Mapping, Forecasting, Network Assessment and Community Outreach for Infectious Disease
The Realtime Global Biosurveillance Platform for Mapping, Forecasting, Network Assessment and Community Outreach for Infectious Disease will monitor, translate and codify global reporting of infectious disease in 65 languages in realtime. GDELT already recognizes an ever-growing inventory of global diseases and driving factors like water, food and health access, evolving narratives and emotional responses bearing on disease vulnerability, captures coverage of transit routes and human-animal interactions and couples this with 21 billion words of socio-cultural academic literature spanning 70 years and a news backfile spanning four decades covering the base narratives of local communities and their reactions to crisis, as well as global to local influencers for community engagement. Through one of the largest local source monitoring and machine translation programs in the world, GDELT previously provided the first detection of the Ebola outbreak well ahead of previous initiatives and uniquely updates every 15 minutes, offering near-realtime alerting. GDELT uniquely combines disease indicators with the contextual underpinnings that indicate an area’s risk profile for disease outbreak and govern how a disease is likely to spread there, including realtime image-based ground truth assessments of environmental conditions like drought and air quality, societal stressors like conflict and socio-cultural tension, likely societal response based on 70 years of academic, NGO and governmental reporting on socio-cultural factors, and the communicative strategies most likely to assist in countering misinformation and changing health practices. This realtime biosurveillance platform will offer a powerful resource for global infectious disease, monitoring risk factors, providing realtime alerts, mapping ongoing outbreaks, contextualizing them to provide risk indicators and providing communicative strategies to help manage them.
At its core, the platform focuses on how to leverage the vast volumes of existing data on both disease outbreaks and, most critically and uniquely, the precursor physical and societal stressors that increase disease risk, establishing one of the first central analytic infrastructures that can collect all of this material together in a single place and translate it from a myriad local sources and languages, apply robust globalized algorithms capable of codifying it all into a single uniform representation and analyze and visualize it in a meaningful and operationally relevant fashion. The majority of this knowledge lies in non-traditional repositories, from local news media in local languages to academic literature and NGO/governmental archives to millions of reports scattered across the open web. GDELT represents one of the largest open data initiatives tackling these challenges, making it both the ideal platform upon which to monitor global disease risk and entirely novel among current approaches. The rich socio-cultural context of GDELT’s 70-year socio-cultural knowledgebase and 40-year news knowledgebase, coupled with its realtime narrative and emotional dimensions make it possible to move beyond simple tactical response to more strategic targeting of the underlying socio-cultural drivers and enabling forces undergirding disease outbreak.
Unlike previous initiatives which have focused nearly exclusively on disease-centric monitoring, GDELT is a global monitoring program that assesses all elements of the planet in realtime. This uniquely allows it to situate emerging disease risk indicators in context with an especial focus on socio-cultural indicators. For example, it couples textual and photographic indicators of drought and food security with emerging narratives criticizing government health efforts and general suspicion of government intervention (such as suspicion of government-run vs NGO-run health clinics), societal narratives and the key bridges and influencers driving those narratives and conversations.
In the area of biosurveillance there have been numerous past attempts to build global monitoring platforms. However, previous systems A) still rely on human coding for key components, making them unsuitable for realtime monitoring, B) have minimal reach into local sources and languages around the world, preventing them from detecting outbreaks until long after they have reached critical stage and are already identified locally as a crisis, C) merely extract disease incidents without the surrounding narrative and emotional context to gauge evolving local and global reaction to the outbreak, D) monitor only disease, preventing a whole-of-earth understanding of social and physical contagion conditions, E) cannot access the rich existing socio-cultural literature on affected areas to estimate the most effective narrative and social counter contexts, F) are unable to access or process ground truth imagery to gauge true ground conditions in realtime, G) are unable to unpack or identify shifts in the baseline emotional and narrative discourse caused by the outbreak, and finally H) lack the deep analytics and computational expertise to leverage all available global data to tease out the earliest indicators and evolution of outbreaks in realtime at global scale. GDELT is perhaps the only global monitoring platform today that brings all of these capabilities together to bear on society’s most pressing issues from conflict to wildlife crime, natural disasters to disease outbreak.
The extremely limited reach of current biosurveillance efforts has been a critical point of failure. For example, one heavily used biosurveillance platform's ability to detect disease outbreaks at early stages is severely constrained by its very limited sourcing, which are overwhelmingly dominated by English language Google News RSS feeds. Over the last four months Google News RSS feeds have accounted for almost 70% of that platform's news content, of which just 36% was not in English and just 12% was from languages other than the big five. That platform thus primarily reflects Western media attention, rather than local events and is one of the reasons it failed to catch the Ebola outbreak until after it had garnered international headlines. Similarly, practitioner systems are limited to actual disease outbreaks or warning signs and are entirely blind to the leading edge socio-cultural and non-disease stressors that provide the most robust and longest-horizon warning signs. In short, current systems are largely limited to primarily Western visibility of disease outbreak and are almost exclusively focused on disease occurrence, making them largely historical postmortem records, rather than true biosurveillance systems proactively identifying conditions and narratives deteriorating towards those ideal for disease outbreak.
The proposed system attempts to correct these limitations of the current biosurveillance landscape to catalog worldwide disease outbreak as it is reported, coupling it with GDELT’s socio-cultural knowledgebase to offer four key biosurveillance dashboards. A realtime map will update every 15 minutes, reflecting the current state of global activity, while realtime alerts will flag emerging situations and detected patterns as they are reported. Geographic analyses highlight emerging patterns, hot zones, and induced transit corridors, along with local reaction, allowing for realtime alerts. The map will also serve dual-use as a public engagement tool, with a public-friendly dashboard reporting realtime outbreaks at vastly higher resolution and reach than previous solutions. Influencer diagrams map the connections among people, organizations, topics, and geographies, pinpointing key influencers and vulnerabilities. Media influence networks identify key stakeholders to maximize messaging to key constituencies and communities. Realtime photographic monitoring of all available news imagery emerging from each area offers ground truth assessment of environmental and health conditions. The end result will be one of the world’s first realtime platform for visualizing, modeling, assessing and understanding global disease outbreak in context.
The first dashboard, aimed at citizens and journalists is a realtime interactive map updating every 15 minutes, placing a dot on the map at each location associated with disease in media reporting. Clicking on a location will display a list of all worldwide coverage mentioning that location in a disease context. Concerned citizens could use this map to see how their own community is affected by and contributes to disease vulnerability and current outbreaks and the map could eventually support citizen-reporting overlays. It will also enable rich public outreach, such as localized “disease vulnerability near me” interactive exhibits and informational campaigns that are able to reach far beyond existing solutions' limited Western focus. Journalists can use it to illustrate the extent of current outbreaks or to provide context to stories by finding past incidents from the same location and understand key risk factors. An animated version of the map will also be created, showing how disease trends are changing over time. The map will also be available as a GeoJSON API compliant with CartoDB’s synchronized layers, allowing anyone to mash up this layer with their own maps of other datasets.
The second dashboard is aimed at communicators and synthesizes all of the monitored coverage into an analytic interface that allows one to explore the global communicative landscape surrounding disease. It automatically compiles a list of top news outlets, authors, organizations, names, locations and subtopics appearing in each disease area. Combined with textual and topical search, it allows a user to search for “Zika in Florida” and get back a list of the top news outlets and reporters worldwide covering it, as well as the common angles, framings and emotional language they use to describe it. For example, are outlets recommending dangerous or misleading health advice that should be corrected and who would be the best reporters to engage with?
The third dashboard offers an environment similar to the communicative dashboard, but focuses instead on the networks of people, organizations, locations and topics connected through the content of all that coverage. Taking the example of a search for “Ebola in Guinea,” this dashboard will compile a list of every major figure, organization and location mentioned in all matching coverage and the connections among them captured in those articles. Over several years of pilot work ranging from environmental issues to conflict spaces, such networks have been found to be creatable in a matter of seconds, yet closely approximate the macro level contours of human expert-assessed networks taking many weeks or months to compile. Moreover, unlike human assessment, which typically only captures explicit connections, these machine induced networks additionally capture latent (hidden) connections in which specific connections become visible only when looking at the scale of tens of thousands of articles. In the case of disease outbreak, such geographic networks offer longitudinal and realtime views into the population movement corridors critical to understanding disease spread.
Finally, the fourth dashboard situates the news-based insights of the first three dashboards within 70 years of historical socio-cultural academic literature and NGO/governmental reporting. Outbreaks do not occur in a vacuum – a rich tapestry of economic and environmental stressors, cultural practices, beliefs, customs, history, views and narratives all contribute to outbreak vulnerability. Awareness and understanding of such socio-cultural underpinnings plays a critical role in developing effective counter campaigns that speak directly to local narratives. The inclusion of a citation graph over the collection makes it possible to perform “find an expert” searches to identify the most heavily cited experts specializing in cultural practices influencing disease spread in an area.
The combination of these four dashboards will offer an unprecedented biosurveillance platform for visualizing, communicating, analyzing and contextualizing global disease risk and spread.