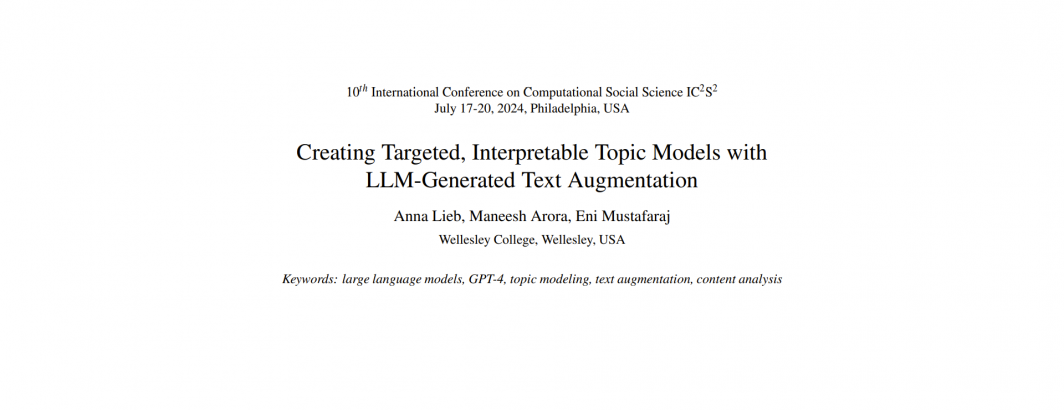
Making sense of natural language text at scale is a common challenge in computational social science research, especially for domains in which researchers have access to an abundance of unlabeled data but face a shortage of labeled data. Unsupervised machine learning techniques, such as topic modeling and clustering, are often used to identify latent patterns in unstructured text data in fields such as political science and sociology. These methods overcome common concerns about reproducibility and costliness involved in the labor-intensive process of human qualitative analysis. However, two major limitations of topic models are their interpretability and their practicality for answering targeted, domain-specific social science research questions. In this work, we investigate opportunities for using LLM-generated text augmentation to improve the usefulness of topic modeling output. We use a political science case study to evaluate our results in a domain-specific application.