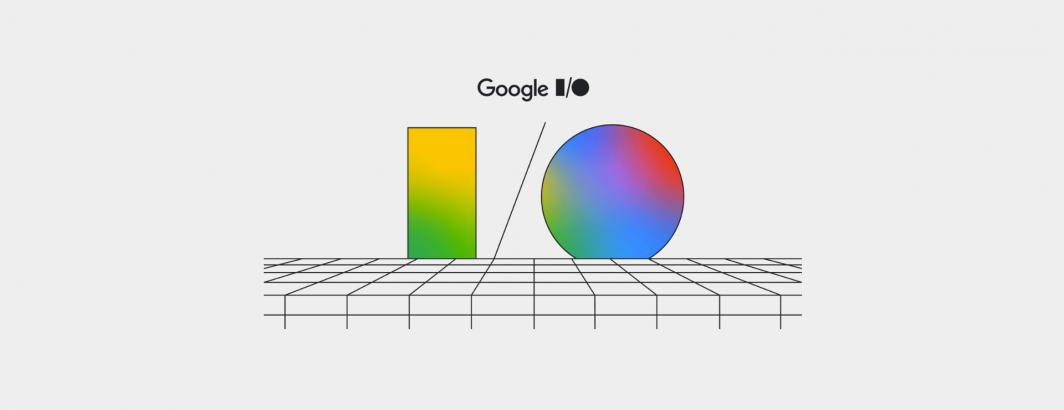
One of the many interesting themes of Google I/O 2024 was just how many attendees on the sidelines were predicting the end of RAG as Google announced early access to its 2M token Gemini model and again teased a 10M token version and the eventual availability of unlimited context windows. Perhaps most fascinating of all was the sheer number of attendees and startups I met who noted that their entire available corporate archives that they thought they might ever analyze with an AI model already fit comfortably within Gemini's existing 1M token model. A constant theme was "Who needs RAG in a world where everything fits natively in the context window?" Yet, this reflects both a failure of imagination in how GenAI models will be applied in the future and just how massively data-intense GDELT's vision of cataloging and understanding the planet truly is.
While context windows continue to expand, there are a number of technical challenges and capability tradeoffs to ever-larger context windows. While today's 1M token models like Gemini 1.5 Pro can indeed process a million tokens of input at once, we find that in practice their attention and reasoning capabilities have not scaled with their context windows, meaning that when pressed to their max window sizes, their performance tends to drop substantially. For example, RW-NIAH needle in a haystack tests on Gemini 1.5 Pro show extremely poor performance on real world content not found in its training data, demonstrating that for many tasks simply fitting all of the content in the context window is no substitute for prefiltering and reformatting for the model. In other words, even in cases where all data fits within the context window, RAG still offers powerful advantages in distilling down the input to just the most relevant entries to help the model focus.
Until unlimited context windows become both widely available and robust, RAG will still be an absolute requirement for data-intensive applications like GDELT simply because the sheer volume of GDELT's daily monitoring volume means no currently contemplated context window sizes would be sufficient to examine even a small fraction of GDELT's monitoring. Critically, even limitless context windows will still require RAG-like solutions at GDELT scale, simply because even scaled attention cannot fit the entirety of all global reporting.
Thus, far from dead, RAG will continue to play a crucial role in enabling GDELT scale classes of AI-powered analytics.