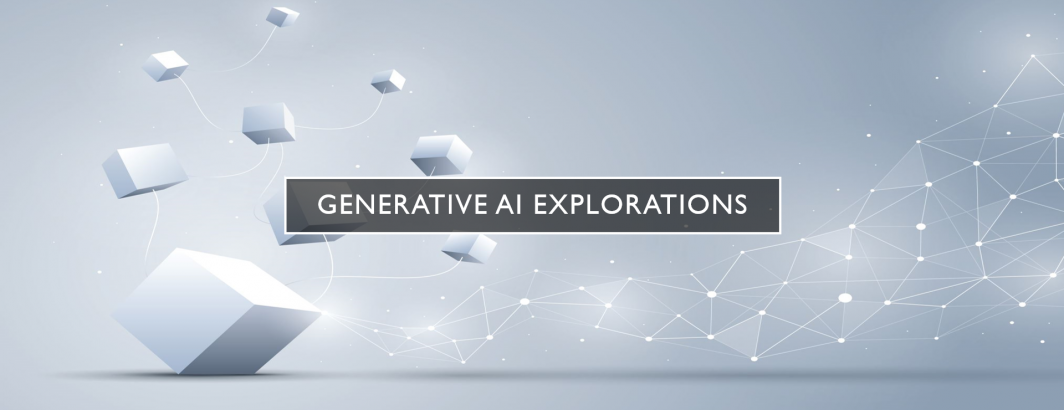
One of the more remarkable reminders of the Wild West state of Generative AI came in a recent meeting I attended where a contractor was pitching its video analytics services and made the astonishing claim that its open source pipeline of Whisper ASR and Tesseract OCR achieved 99.9999% (yes they claimed four nines) "accuracy" at processing global video content. In keeping with today's standard GenAI tech hype cycle, the technical SMEs in the room raved about their own experiments with Whisper and Tesseract and how excited they were about AI in general. In my role as senior technical advisor to the decision makers in the room, I alone asked the question that not one of the myriad technical experts assembled in the room thought to ask: how exactly did they measure that accuracy? More to the point, as the only one in the entire room who had actually applied those tools at scale to global video content under rigorous production standards, how were they able to achieve accuracy levels orders of magnitude beyond anything we've observed in the real world?
The answer?
They achieved 99.9999% accuracy because that was the percentage of airtime for which one or both of the tools generated at least one character of output.
Bizarrely, not one of the technical SMEs in the room paused in their effusive praise of generative AI for just a solitary instant to catch the existential falsehood of that statement. In fact, when I clarified my question and asked how they measured "accuracy" and not "output", this room full of self-proclaimed applied generative AI luminaries suggested I simply had no understanding of statistics or machine learning or AI of any kind to not understand that output equated accuracy.
Without saying a word, I pulled out the raw output files from a few of our myriad Whisper and Tesseract demos, where the outputs were complete gibberish that did not reflect to a single character the actual spoken or written words of the clip and asked, would this be considered 100% accuracy under their rubric, since there was output for every second of airtime.
Suddenly the room fell quiet. The previously hard-selling presenters lowered their voices to just above a whisper and confirmed that, yes, under their rubric those examples would be 100% "accurate" and that perhaps "alternative metrics might be useful under certain conditions." They then argued that generative AI systems shouldn't be held to the same standards as previous tools because "failure is simply baked into generative AI so it isn't fair to measure it." Yes, you read that right – a contractor actually argued in front of an entire room that it isn't fair to measure the accuracy of generative AI tools.
As seems to be all-too-often the norm these days among the GenAI developer community, the technical SMEs in the room resumed arguing that output equals accuracy and that traditional accuracy metrics shouldn't apply to generative AI systems. Thankfully, in this case as the senior advisor in the room my voice held sufficient weight to convince the decision makers to request that the contractor come back with actual traditional accuracy metrics comparing on a set of actual videos from the decision makers' organization the output of their tool versus hand transcribed gold spoken and written text transcripts.
It is a deeply unfortunate reflection of the generative AI hype cycle that entire rooms of GenAI technical SMEs can agree in unison in 2024 that "output equals accuracy" and that it isn't "fair" to measure the true accuracy of GenAI systems. Unfortunately, this experience is far from an outlier these days, especially in governmental circles, offering a critical reminder to organizations to bring in critical experts who deeply understand the limitations of these systems and have deep experience with applying them in the real world to help them understand their applicability to their own needs and to pierce through the hype and hyperbole to assess these tools through traditional gold standard accuracy tests.