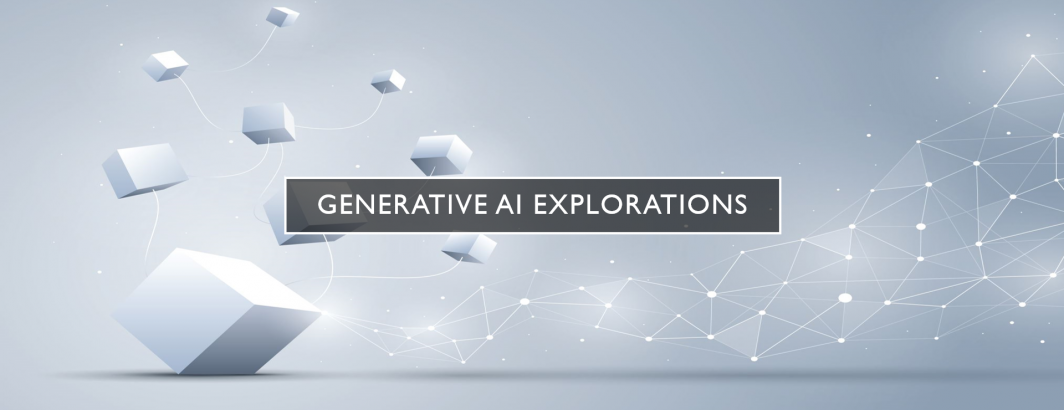
One of the most underappreciated aspects of AI trust and safety efforts is the critical importance of bringing in external experts who are not just outside of the company building the LLM, but outside of the core trust and safety community itself – applied SMEs rather than T&S SMEs. Perhaps the single most striking aspect of our work with the generative AI community over the past few years and especially over the past year and a half is the sheer volume of P0's we uncover each week in the world's most-used GenAI systems, with many of these issues having been a dedicated focus of massive red teaming efforts bringing together some of the world's most recognized names in AI T&S, vast armies of academics and red teamers and some of the top SMEs in those specific subcategories. Given the sheer investment and star power devoted to many of the areas we uncover P0's in each day, how could we be uncovering this many existential issues? The answer: because the GenAI community today is focusing too heavily on a cottage industry of T&S & SME expertise suffering from increasingly narrow groupthink for its reviews and not nearly enough on those power users who are applying these models to real world content under real world conditions to answer real world questions in the real world.
As but one example, we were recently testing the advanced analytic and reasoning capabilities of a top LMM. In the course of our testing, we uncovered something strange: our test suites flagged a troubling behavior – when asked whether a given image was a deep fake or had been altered in any way, it would systematically flag as deep fakes real images depicting certain politicians or topics, while systematically reporting as completely authentic legitimate deep fakes of other politicians and topics. Worse, it would hallucinate that it had run various real-world deep fake and alternation detection software packages and report various actual metrics from those tools – none of which it had run on the image, but which made its prognostications all the more authentic-seeming and frightening. In short, take the latest very real viral negative social media image about politician A and ask the model whether it is real and it will answer definitively and with copious evidence that the image was faked, while asking about an actual deep fake of politician B will yield an answer that the image is entirely authentic and not faked – with grave implications to elections and maintaining an informed electorate.
In conversations with this company after reporting this P0, who to their credit escalated it immediately as a show-stopping issue, we learned much to both our and the company's surprise that this exact scenario had been the subject of massive red teaming that had brought together a star-studded cast of academics, consultants, SMEs and T&S experts who had eventually signed off that this scenario could never happen based on all of their work. Yet, it took us less than 60 seconds to identify it in our most basic test suite. What explains this?
As we dove further into this issue with the company and examined the actual prompts and scenarios that had been examined by all these luminaries in their reports, we found something fascinating: for all their trust and safety, disinformation and elections expertise, none of them had thought to test what would happen if a user asked whether an image was a deep fake for different politicians and topics, having wrongfully assumed that the narrow set of prompts they tested were representative of the model's entire performance on the topic – an oversight that any genai T&S expert should have been only all too familiar with.
So what explains this?
The answer is that too much of the red teaming and AI T&S efforts today are being left to a community that is increasingly suffering from groupthink and increasingly examining models along similar kinds of adversarial lines of reasoning that leave the resulting safety mitigations exceptionally brittle and reflective of a very specific way of thinking.
How do we fix this?
While companies should continue to engage with the AI T&S community, continue to collaborate with academics and continue to work with SMEs, for AI systems to truly begin to address the deeper issues that plague current generative models, they must engage far more closely with their applied user communities – those who are actually using these models in the real world and see how they actually perform each day on content that represents the full rich diversity of out entire planet.