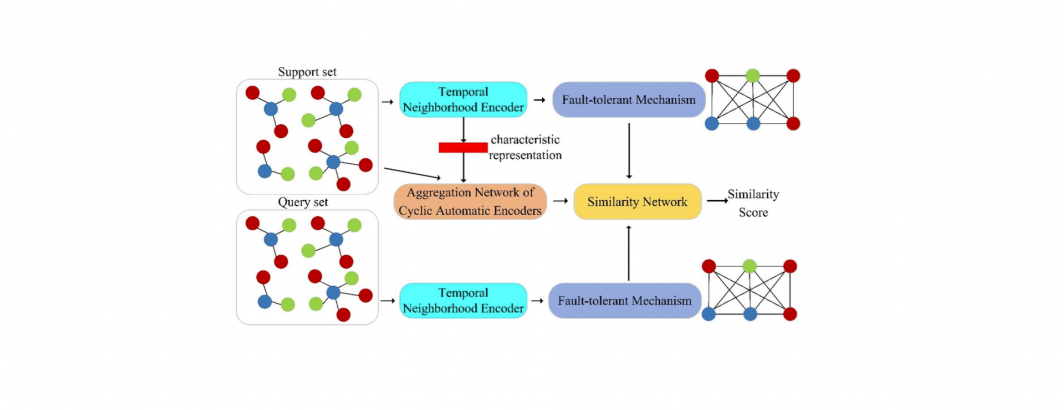
Traditional knowledge graph completion mainly focuses on static knowledge graph. Although there are efforts studying temporal knowledge graph completion, they assume that each relation has enough entities to train, ignoring the influence of long tail relations. Moreover, many relations only have a few samples. In that case, how to handle few-shot temporal knowledge graph completion still merits further attention. This paper aims to propose a framework for completing few-shot temporal knowledge graph. We use self-attention mechanism to encode entities, use cyclic recursive aggregation network to aggregate reference sets, use fault-tolerant mechanism to deal with error information, and use similarity network to calculate similarity scores. Experimental results show that our proposed model outperforms the baseline models and has better stability.