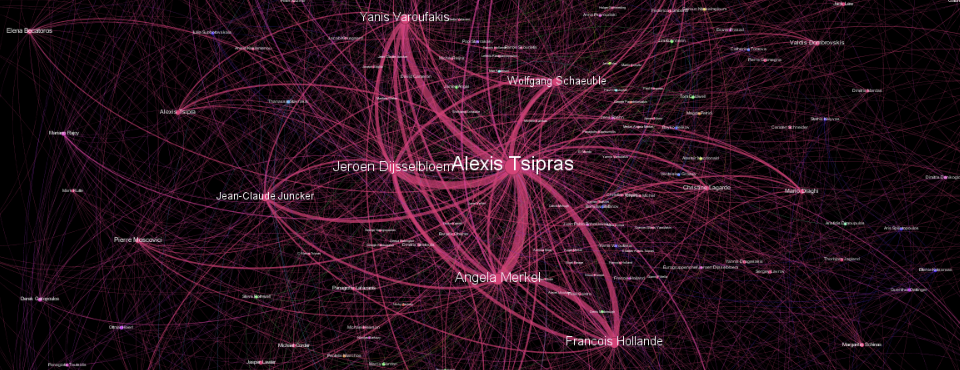
To reason about the world we must first be able to represent it. Machines, however, see the visual and textual worlds very differently, making it profoundly difficult to reason about the world when looking across their distinct lenses.
Computational representations form the lens through which we conceptualize the very nature of how news encodes the world around us and how we as societies understand the world through that representation and the interaction and interplay between news and the functioning of society.
To date, GDELT has analyzed more than two billion global news articles spanning 30 years in 150 languages, half a billion global news images and a decade of television news, translating content in realtime and identifying events, narratives, emotions, claims and relationships.
To do all of this, GDELT relies upon an ensemble of classical grammatical, statistical, traditional machine learning and deep learning approaches, including Google Cloud’s Vision, Video, Speech-To-Text, Natural Language and Translation APIs, together with myriad non-neural algorithms.
The end result is a divergent landscape of fixed tag-based taxonomies describing the visual world and open-ended entity lists and parse trees capturing the textual one.
Actionable tasks like question answering over the news, automated detection of emerging events from wildlife crime to civil unrest, identifying misinformation, contested and inorganic narratives and even forecasting societal-scale events requires reconciling these very different representations, together with classical representations in a way that permits unified multimodal visual-textual reasoning.
How do we move from the metadata tags, entity lists, tabular scores, similarly matching and parse trees that define today’s production machine learning representations towards the globally contextualized high-order facts, relationships, narratives and emotions that allow us to answer real-world questions?
In particular, conceptualizing news stories as embedded in a fluid global network of information and tracking how those stories evolve, fold and seed other stories as their contours constantly evolve, dissolve and reform, requires reimagining how we represent and reason across our increasingly multimodal world and moving beyond entity lists, category tags and keywords towards meaningful semantic encodings from which machines can begin to abstract actionable insights about the functioning of our societies.
Given the centrality of these needs, you will see a flurry of developments from GDELT over the coming months as we explore how the field might move beyond merely representing information and beyond our divergent representations towards actually reasoning over chaotic, conflicting realtime multimodal streams to answer society’s most pressing grand challenge questions, including forecasting the very future of our shared world.