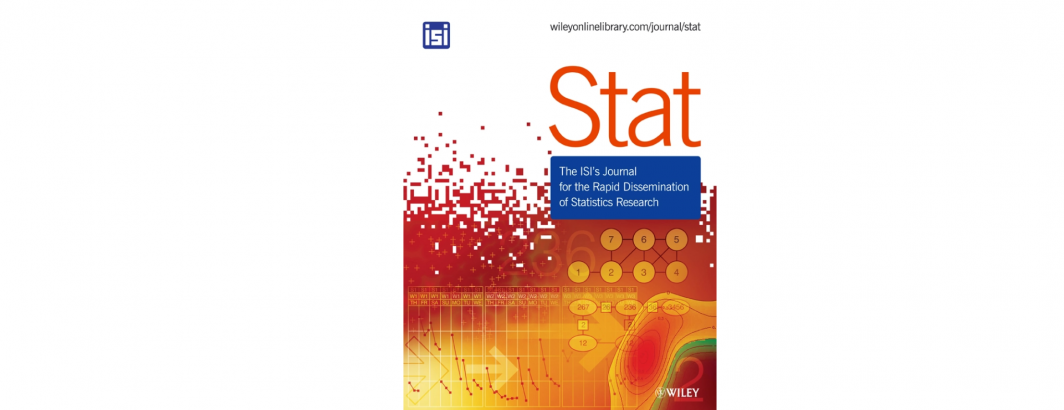
This paper by two researchers at Fudan University explores tensor completion:
Tensor completion is among the most important tasks in tensor data analysis, which aims to fill the missing entries of a partially observed tensor. In many real applications, non‐Gaussian data such as binary or count data are frequently collected. Thus it is inappropriate to assume that observations are normally distributed, and formulate tensor completion with least squares based approach. In this article, we develop a new tensor completion method called ExpCP for non‐Gaussian data from the exponential family distributions. Under the framework of penalized likelihood estimation, we assume the tensor of latent natural parameters admits a low‐rank structure, and further induce regularization by integrating auxiliary information. Moreover, we propose an efficient algorithm based on the alternating direction method of multipliers to solve the optimization problem. We establish the algorithmic convergence results, and demonstrate the efficacy of our proposal with comprehensive simulations and real data analysis on GDELT social events study. The empirical results show that our method offers improved modeling strategy as well as efficient computation.