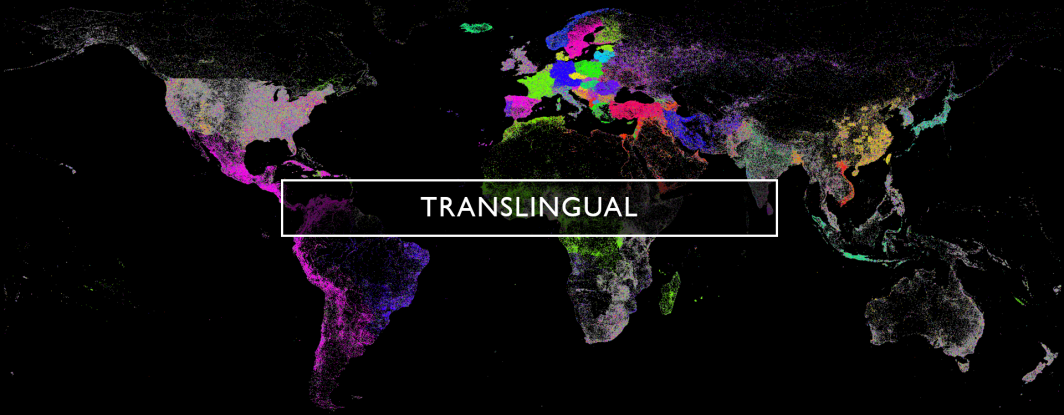
When GDELT Translingual first debuted in February 2015, we ensured that its models were able to translate every word in the GKG thematic categories and GCAM's emotional categories. Given the inclusion of a majority of human and zoonotic diseases in the GKG thematic taxonomies, this meant that we were able to recognize and accurately translate the majority of disease mentions across the 65 languages covered by Translingual. Little did we think when we were creating these models throughout 2014 that half a decade later they would be used to send out one of the very first alerts of Covid-19. It was only because we had gone to great lengths to ensure the entire GKG was covered by our translation models that we were able to pick up those early mentions of "SARS-like viral pneumonia of unknown origins" in Chinese-language press, despite pneumonia not being a common word appearing in high quantities in the news when we trained our models.
Fast forward to today and we are seeing GDELT deployed in almost every imaginable field of study that require its models to handle everything from the arcane medical jargon of pandemic coverage to the complex scientific language of climate change to the novel words of cybersecurity to the infrequently used words of international diplomacy and human rights law to mentions of obscure animal and plant species in wildlife crime. These emerging applications require faithful translation of the far corners of the world's languages, delving heavily into rare constructs, uncommon, obscure and specialty terminology and loan words and ideographs.
Prior to January 2020, the medical and scientific terminology of the Covid-19 pandemic almost never appeared outside specialty industry-specific newsletters, whereas today even small local news outlets run articles that look more at home in a medical journal than a mainstream small town newspaper. Similarly, the deeply technical terms of cybercrime and cyberwarfare were once rarely seen outside academic and industry publications, yet they too appear with ever-increasing regularity. In the same fashion, the topics and terms rarely seen in the news today may similarly become commonplace without warning.
Traditional approaches to constructing machine translation models through vast quantities of bilingual and monolingual corpi are insufficient for faithfully capturing these far edges of the linguistic landscape, yet it is critical that our models capture this language properly so that we are able to flag the earliest glimmers of tomorrow's biggest stories.
Towards this end, Translingual 2.0 has a dedicated focus on incorporating these far corners of the linguistic landscape and we are applying a number of approaches to specifically search for the underrepresented corners of each language, especially rare and loanwords and uncommon constructs and building training pipelines that ensure they are incorporated into our models despite the high imbalance between their sporadic use and the far more common constructs of each language.