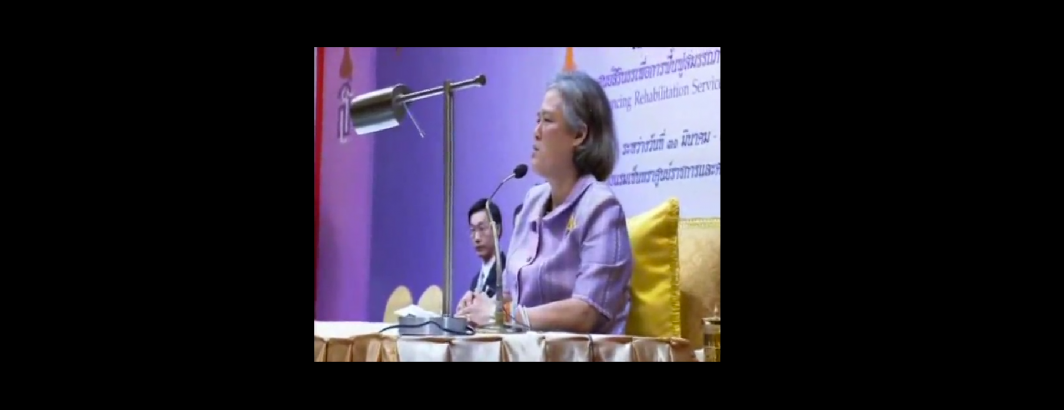
While Large Multimodal Models (LMMs) are increasingly positioned as replacements for the full range of classical AI systems like OCR, ASR, NLP, NLU and CV tasks, the reality is that their extreme instability and consistent hallucinations are existential challenges that render them unsuitable for real-world production use cases like OCR. Throughout all of our OCR experiments to date, we have consistently found LMMs to alternate between extremely high accuracy approaching classical systems, often with superior structure recovery, and catastrophic failures that hallucinate text that isn't present in the image, exclude huge swaths of the image's text, randomly drop text blocks and exhibit such instability that ten runs in a row might yield ten very different results.
We continue to run regular benchmark tests of classical OCR systems, both open source like Tesseract and EasyOCR, and SOTA commercial systems like GCP's Cloud Vision API and emergent approaches like LMMs and have yet to find a solution that is as robust as SOTA commercial systems like Cloud Vision when deployed at scale under real world conditions in real life production workflows. These findings mirror what we continue to find with the GenAI landscape overall: tremendous hype and a steady flood of mediagenic and heavily hyped one-off demos, but existential limitations that negate their ability to power fault-sensitive production workflows in the real world.